Crunching the Numbers: Easy Statistical Treatments for Your Research

Ever stared at a pile of data and thought, "What now?" You're not alone. Research can be a maze, especially when numbers get involved. But here's the thing: statistical treatment isn't just for math whizzes. It's a tool that helps make sense of data, guiding you through the research labyrinth. In this article, we'll break down how statistical treatment in research works, why it's important, and how you can use it without losing your mind.
Key Takeaways
- Statistical treatment helps transform raw data into meaningful insights.
- Choosing the right statistical method is crucial for clear results.
- Balancing complexity with simplicity ensures your research is understandable.
- Reproducibility in statistical treatment boosts the credibility of your research.
- Understanding and interpreting statistical results is key to successful research.
Understanding the Role of Statistical Treatment in Research
Defining Statistical Treatment
Statistical treatment involves the application of statistical methods to collect, analyze, and interpret data. Imagine you're trying to figure out if a new teaching method is effective. You'd gather data from students who used the method and those who didn't, then apply statistical techniques to see if there's a significant difference in their performance. This process helps researchers make informed decisions based on data rather than guesswork.
Importance in Research Methodology
In research, statistical treatment is like the backbone that supports your entire study. Without it, your findings might just be random noise. It ensures that the results you get are reliable and not just a fluke. Think of it as using WhatsApp to communicate quickly and efficiently; statistical treatment helps convey the 'message' of your data clearly and accurately.
Common Misconceptions
Many people think statistical treatment is just about crunching numbers, but it's way more than that. It's about understanding what those numbers mean in the real world. Some folks believe it's only useful for large datasets, but even small studies can benefit from proper statistical analysis. Others might think it's too complicated, but with the right tools and guidance, anyone can grasp the basics.
Designing Effective Statistical Treatments for Research Studies
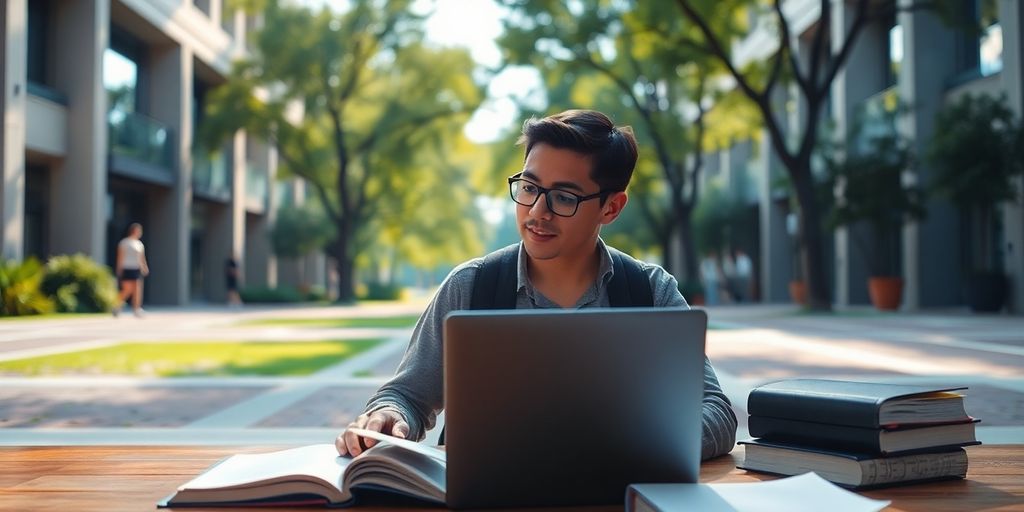
Choosing Appropriate Statistical Methods
Selecting the right statistical methods is like picking the right tool for a job. It's about understanding your research questions and the type of data you have. Choosing the wrong method can lead to misleading results. For instance, if you're analyzing survey data, you might consider logistic regression if your outcome is binary, or ANOVA if you're comparing means across groups. The choice should align with your study's goals, ensuring that the methods are robust enough to handle your data's complexity without oversimplifying the findings.
Balancing Complexity and Clarity
Research often walks a fine line between complexity and clarity. On one hand, sophisticated models can capture intricate data patterns, but they can also obscure the main findings if not presented clearly. It's crucial to balance these elements to make your research accessible. Here are a few tips:
- Simplify your language to explain complex models.
- Use visual aids like graphs and tables to illustrate key points.
- Focus on the main findings rather than getting lost in technical details.
Ensuring Reproducibility
In the world of research, reproducibility is king. It means others can follow your methods and arrive at the same results, which is essential for building trust and validating findings. To achieve this, document every step of your analysis process, from data collection to statistical testing. Use software tools that support reproducibility, such as R or Python, which allow you to share your code and data openly. This transparency not only strengthens your research but also aligns with practices advocated by platforms like LinkedIn, where professionals share insights and methodologies.
Incorporating these elements into your research design can not only enhance the quality of your study but also make the daunting task of "how to write your thesis in 30 days" a bit more manageable. By focusing on these core principles, you can ensure that your research is both rigorous and impactful.
Analyzing Data: Statistical Treatment Techniques
Descriptive vs. Inferential Statistics
When it comes to analyzing data, understanding the difference between descriptive and inferential statistics is key. Descriptive statistics provide a way to summarize and present data in a meaningful way, often using measures like mean, median, and mode. They help in giving a clear picture of what the data looks like. On the other hand, inferential statistics allow researchers to make predictions or inferences about a population based on a sample of data. This involves using data from a small group to make generalizations about a larger group. For example, if you survey 100 people about their favorite ice cream flavor, you might use inferential statistics to guess what the favorite flavor is for all people in a city.
Utilizing Software for Data Analysis
In today's digital age, using software for data analysis is almost a given. Programs like SPSS, R, and Python libraries such as Pandas and NumPy offer powerful tools for crunching numbers and visualizing data. These tools help in automating complex calculations, creating visualizations like graphs and charts, and even running simulations. They are essential for handling large datasets that would be impossible to analyze manually. The choice of software often depends on the complexity of the analysis and the user's familiarity with the tool.
Interpreting Statistical Results
Interpreting the results of statistical analysis is where the art meets the science. It's not just about understanding the numbers but also about what they mean in the context of your research. For instance, a p-value in a hypothesis test tells you whether your results are statistically significant. But it's equally important to consider the practical significance—does the result make a real-world difference? Misinterpretation can lead to incorrect conclusions, so it's crucial to carefully analyze and question the results. This guide on empirical analysis highlights the importance of considering context and variables to avoid misinterpretation.
Challenges in Applying Statistical Treatment in Research
Addressing Data Variability
When dealing with data, one of the biggest hurdles is its inherent variability. Data points can fluctuate, sometimes wildly, due to numerous factors like measurement errors or random sampling differences. This variability can obscure the true patterns researchers are trying to identify. For instance, a dataset gathered from Instagram might show trends that are more reflective of user engagement patterns rather than the actual phenomena being studied. Understanding and managing this variability is crucial to ensure that the statistical analysis remains robust and reliable.
Overcoming Sample Size Limitations
Sample size is another critical aspect that can significantly impact the outcomes of statistical treatments. A small sample size might not adequately represent the population, leading to skewed results. Researchers often face challenges in obtaining a large enough sample due to constraints like budget, time, or accessibility. To mitigate these issues, researchers might employ techniques like bootstrapping or use statistical software to simulate larger sample sizes, thereby enhancing the credibility of their findings.
Mitigating Bias and Errors
Bias and errors are persistent challenges in any research involving statistical treatment. Whether it's selection bias, where the sample isn't representative of the population, or measurement errors that skew data, these issues can lead to incorrect conclusions. Researchers must be diligent in designing their studies to minimize these biases. This might involve using random sampling methods, ensuring instruments are calibrated correctly, or employing blinding techniques in experimental designs. Acknowledging these biases and actively working to reduce them can significantly improve the quality of the research findings.
Enhancing Research Validity Through Statistical Treatment
Ensuring Statistical Significance
Statistical significance is like the backbone of research validity. It's about deciding if your findings are not just random flukes. Imagine you're flipping a coin, and it lands on heads ten times in a row. Is the coin rigged, or was that just luck? Statistical significance helps you figure that out. In research, a small p-value usually indicates that your results are statistically significant, meaning they're less likely to be due to chance. So, when you're conducting a study, it's crucial to set a threshold for this p-value to help determine the reliability of your findings.
Improving Confidence Intervals
Confidence intervals give you a range within which you can say, "I'm pretty sure the true value lies here." It's like saying, "I think the average height of students in this class is between 5'6" and 5'8"." This range helps to understand the precision of your estimate. A narrower confidence interval means more precision, while a wider one suggests more uncertainty. When you improve your confidence intervals, you're essentially tightening that range, which boosts the trustworthiness of your conclusions.
Generalizing Findings
Generalizing is about applying your study results to a broader population. Let's say you did a study on the effects of a new teaching method in one school. Can you say it'll work in all schools? Not necessarily. You need to ensure your sample is representative of the larger group you're interested in. This is where random sampling comes in handy. By choosing a sample that accurately reflects the population, you can make more confident generalizations. However, be cautious—differences in demographics or settings can affect how applicable your findings are elsewhere.
Incorporating these statistical treatments effectively can significantly enhance the validity of your research. They help ensure that your findings are not only accurate but also applicable to real-world scenarios, thus making your research more impactful.
Case Studies: Successful Statistical Treatments in Research
Healthcare Research Applications
In healthcare, statistical treatments are vital for interpreting complex data. Consider a study examining the impact of a new drug on blood pressure. Researchers might use a randomized control trial to assess the drug's effectiveness. By applying statistical methods like ANOVA (Analysis of Variance), they can determine whether changes in blood pressure are significant or just due to random variation. Such studies often reveal how statistical methods can uncover meaningful insights, ensuring that treatments are both effective and safe for patients.
Social Sciences Methodologies
In social sciences, statistical treatments help in understanding human behavior. For example, a study on the effects of educational interventions on student performance might employ regression analysis. This method helps in identifying the relationship between variables, such as teaching methods and student success. Regression analysis can highlight which interventions are truly beneficial, allowing educators to make informed decisions. This approach underscores the importance of statistical tools in developing effective educational strategies.
Innovations in Data Science
Data science has revolutionized how we approach research. In recent years, the integration of machine learning with statistical techniques has opened new possibilities. For instance, predictive modeling in data science uses algorithms to forecast outcomes based on historical data. This is particularly useful in fields like finance and marketing, where predicting consumer behavior can lead to better business strategies. The synergy between machine learning and statistics is creating innovative pathways, showcasing how statistical treatments are evolving in the age of big data and AI. This evolution is deeply tied to AI's value creation, which is shaped by its underlying philosophy.
Future Trends in Statistical Treatment for Research
Emerging Statistical Techniques
In recent years, new statistical methods are popping up that offer fresh ways to analyze data. These techniques are not just fancy add-ons; they actually help researchers handle complex data sets better. One emerging method is Bayesian statistics, which lets researchers update their findings as new data comes in, making the results more dynamic and relevant.
Impact of Big Data on Research
Big data is everywhere now, and it's changing how we do research. With so much information available, researchers can ask bigger questions and get more detailed answers. Big data allows for deeper insights into patterns and trends that were hard to see before. But with this comes the challenge of managing and interpreting vast amounts of data, which requires advanced tools and skills.
Integrating AI in Statistical Analysis
Artificial intelligence is becoming a big player in statistical analysis. AI can help automate complex calculations and uncover patterns that might be missed by human eyes. This integration is not just about speed; it's about accuracy and finding connections in data that were previously hidden. As AI continues to evolve, it promises to make statistical analysis more robust and insightful.
In conclusion, these trends are reshaping the landscape of statistical treatment in research. As we move forward, staying updated with these trends will be crucial for researchers aiming to leverage the full potential of their data.
As we look ahead, the future of statistical methods in research is bright and full of exciting possibilities. New technologies and approaches are changing how we analyze data, making it easier and more effective. If you're curious about how these trends can help you in your studies, visit our website for more information and resources that can guide you through your research journey!
Conclusion
In wrapping up our exploration of statistical methods, it's clear that understanding the basics can significantly enhance your research. You don't need to be a math whiz to grasp these concepts; a bit of patience and practice goes a long way. By breaking down complex data into manageable pieces, you can uncover insights that might otherwise remain hidden. Whether you're working on a thesis or a business report, these tools can help you make informed decisions. Remember, the key is to stay curious and keep questioning the numbers. With the right approach, statistics can be a powerful ally in your research journey.
Frequently Asked Questions
What is statistical treatment in research?
Statistical treatment refers to the methods used to analyze data in research. It helps researchers understand patterns, relationships, and trends in the data they collect.
Why is statistical treatment important in research?
Statistical treatment is crucial because it allows researchers to make sense of their data, draw conclusions, and support their findings with evidence. It helps in making informed decisions based on the data.
What are some common misconceptions about statistical treatment?
A common misconception is that statistical treatment is only for experts. In reality, basic statistical methods can be understood and applied by anyone with a little practice and the right tools.
How do I choose the right statistical method for my study?
Choosing the right method depends on your research question, the type of data you have, and the goals of your study. It's important to understand the strengths and limitations of different methods.
Can statistical treatment help in overcoming sample size limitations?
Yes, statistical methods can help address sample size issues by using techniques that maximize the information gained from a small sample. However, larger samples generally provide more reliable results.
What role does software play in statistical treatment?
Software can make statistical analysis easier by handling complex calculations and providing visualizations. It allows researchers to focus on interpreting results rather than crunching numbers manually.
Crunching the Numbers: Easy Statistical Treatments for Your Research

Ever stared at a pile of data and thought, "What now?" You're not alone. Research can be a maze, especially when numbers get involved. But here's the thing: statistical treatment isn't just for math whizzes. It's a tool that helps make sense of data, guiding you through the research labyrinth. In this article, we'll break down how statistical treatment in research works, why it's important, and how you can use it without losing your mind.
Key Takeaways
- Statistical treatment helps transform raw data into meaningful insights.
- Choosing the right statistical method is crucial for clear results.
- Balancing complexity with simplicity ensures your research is understandable.
- Reproducibility in statistical treatment boosts the credibility of your research.
- Understanding and interpreting statistical results is key to successful research.
Understanding the Role of Statistical Treatment in Research
Defining Statistical Treatment
Statistical treatment involves the application of statistical methods to collect, analyze, and interpret data. Imagine you're trying to figure out if a new teaching method is effective. You'd gather data from students who used the method and those who didn't, then apply statistical techniques to see if there's a significant difference in their performance. This process helps researchers make informed decisions based on data rather than guesswork.
Importance in Research Methodology
In research, statistical treatment is like the backbone that supports your entire study. Without it, your findings might just be random noise. It ensures that the results you get are reliable and not just a fluke. Think of it as using WhatsApp to communicate quickly and efficiently; statistical treatment helps convey the 'message' of your data clearly and accurately.
Common Misconceptions
Many people think statistical treatment is just about crunching numbers, but it's way more than that. It's about understanding what those numbers mean in the real world. Some folks believe it's only useful for large datasets, but even small studies can benefit from proper statistical analysis. Others might think it's too complicated, but with the right tools and guidance, anyone can grasp the basics.
Designing Effective Statistical Treatments for Research Studies
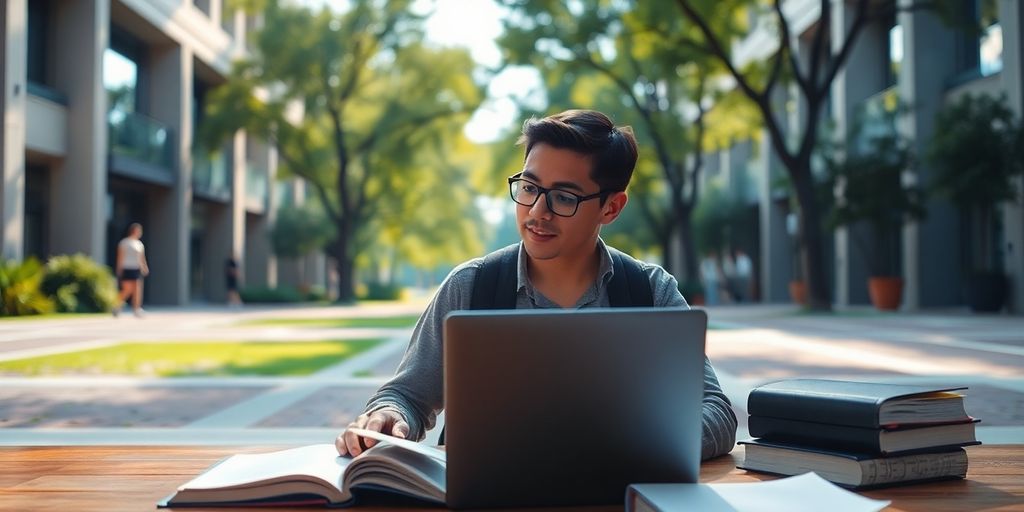
Choosing Appropriate Statistical Methods
Selecting the right statistical methods is like picking the right tool for a job. It's about understanding your research questions and the type of data you have. Choosing the wrong method can lead to misleading results. For instance, if you're analyzing survey data, you might consider logistic regression if your outcome is binary, or ANOVA if you're comparing means across groups. The choice should align with your study's goals, ensuring that the methods are robust enough to handle your data's complexity without oversimplifying the findings.
Balancing Complexity and Clarity
Research often walks a fine line between complexity and clarity. On one hand, sophisticated models can capture intricate data patterns, but they can also obscure the main findings if not presented clearly. It's crucial to balance these elements to make your research accessible. Here are a few tips:
- Simplify your language to explain complex models.
- Use visual aids like graphs and tables to illustrate key points.
- Focus on the main findings rather than getting lost in technical details.
Ensuring Reproducibility
In the world of research, reproducibility is king. It means others can follow your methods and arrive at the same results, which is essential for building trust and validating findings. To achieve this, document every step of your analysis process, from data collection to statistical testing. Use software tools that support reproducibility, such as R or Python, which allow you to share your code and data openly. This transparency not only strengthens your research but also aligns with practices advocated by platforms like LinkedIn, where professionals share insights and methodologies.
Incorporating these elements into your research design can not only enhance the quality of your study but also make the daunting task of "how to write your thesis in 30 days" a bit more manageable. By focusing on these core principles, you can ensure that your research is both rigorous and impactful.
Analyzing Data: Statistical Treatment Techniques
Descriptive vs. Inferential Statistics
When it comes to analyzing data, understanding the difference between descriptive and inferential statistics is key. Descriptive statistics provide a way to summarize and present data in a meaningful way, often using measures like mean, median, and mode. They help in giving a clear picture of what the data looks like. On the other hand, inferential statistics allow researchers to make predictions or inferences about a population based on a sample of data. This involves using data from a small group to make generalizations about a larger group. For example, if you survey 100 people about their favorite ice cream flavor, you might use inferential statistics to guess what the favorite flavor is for all people in a city.
Utilizing Software for Data Analysis
In today's digital age, using software for data analysis is almost a given. Programs like SPSS, R, and Python libraries such as Pandas and NumPy offer powerful tools for crunching numbers and visualizing data. These tools help in automating complex calculations, creating visualizations like graphs and charts, and even running simulations. They are essential for handling large datasets that would be impossible to analyze manually. The choice of software often depends on the complexity of the analysis and the user's familiarity with the tool.
Interpreting Statistical Results
Interpreting the results of statistical analysis is where the art meets the science. It's not just about understanding the numbers but also about what they mean in the context of your research. For instance, a p-value in a hypothesis test tells you whether your results are statistically significant. But it's equally important to consider the practical significance—does the result make a real-world difference? Misinterpretation can lead to incorrect conclusions, so it's crucial to carefully analyze and question the results. This guide on empirical analysis highlights the importance of considering context and variables to avoid misinterpretation.
Challenges in Applying Statistical Treatment in Research
Addressing Data Variability
When dealing with data, one of the biggest hurdles is its inherent variability. Data points can fluctuate, sometimes wildly, due to numerous factors like measurement errors or random sampling differences. This variability can obscure the true patterns researchers are trying to identify. For instance, a dataset gathered from Instagram might show trends that are more reflective of user engagement patterns rather than the actual phenomena being studied. Understanding and managing this variability is crucial to ensure that the statistical analysis remains robust and reliable.
Overcoming Sample Size Limitations
Sample size is another critical aspect that can significantly impact the outcomes of statistical treatments. A small sample size might not adequately represent the population, leading to skewed results. Researchers often face challenges in obtaining a large enough sample due to constraints like budget, time, or accessibility. To mitigate these issues, researchers might employ techniques like bootstrapping or use statistical software to simulate larger sample sizes, thereby enhancing the credibility of their findings.
Mitigating Bias and Errors
Bias and errors are persistent challenges in any research involving statistical treatment. Whether it's selection bias, where the sample isn't representative of the population, or measurement errors that skew data, these issues can lead to incorrect conclusions. Researchers must be diligent in designing their studies to minimize these biases. This might involve using random sampling methods, ensuring instruments are calibrated correctly, or employing blinding techniques in experimental designs. Acknowledging these biases and actively working to reduce them can significantly improve the quality of the research findings.
Enhancing Research Validity Through Statistical Treatment
Ensuring Statistical Significance
Statistical significance is like the backbone of research validity. It's about deciding if your findings are not just random flukes. Imagine you're flipping a coin, and it lands on heads ten times in a row. Is the coin rigged, or was that just luck? Statistical significance helps you figure that out. In research, a small p-value usually indicates that your results are statistically significant, meaning they're less likely to be due to chance. So, when you're conducting a study, it's crucial to set a threshold for this p-value to help determine the reliability of your findings.
Improving Confidence Intervals
Confidence intervals give you a range within which you can say, "I'm pretty sure the true value lies here." It's like saying, "I think the average height of students in this class is between 5'6" and 5'8"." This range helps to understand the precision of your estimate. A narrower confidence interval means more precision, while a wider one suggests more uncertainty. When you improve your confidence intervals, you're essentially tightening that range, which boosts the trustworthiness of your conclusions.
Generalizing Findings
Generalizing is about applying your study results to a broader population. Let's say you did a study on the effects of a new teaching method in one school. Can you say it'll work in all schools? Not necessarily. You need to ensure your sample is representative of the larger group you're interested in. This is where random sampling comes in handy. By choosing a sample that accurately reflects the population, you can make more confident generalizations. However, be cautious—differences in demographics or settings can affect how applicable your findings are elsewhere.
Incorporating these statistical treatments effectively can significantly enhance the validity of your research. They help ensure that your findings are not only accurate but also applicable to real-world scenarios, thus making your research more impactful.
Case Studies: Successful Statistical Treatments in Research
Healthcare Research Applications
In healthcare, statistical treatments are vital for interpreting complex data. Consider a study examining the impact of a new drug on blood pressure. Researchers might use a randomized control trial to assess the drug's effectiveness. By applying statistical methods like ANOVA (Analysis of Variance), they can determine whether changes in blood pressure are significant or just due to random variation. Such studies often reveal how statistical methods can uncover meaningful insights, ensuring that treatments are both effective and safe for patients.
Social Sciences Methodologies
In social sciences, statistical treatments help in understanding human behavior. For example, a study on the effects of educational interventions on student performance might employ regression analysis. This method helps in identifying the relationship between variables, such as teaching methods and student success. Regression analysis can highlight which interventions are truly beneficial, allowing educators to make informed decisions. This approach underscores the importance of statistical tools in developing effective educational strategies.
Innovations in Data Science
Data science has revolutionized how we approach research. In recent years, the integration of machine learning with statistical techniques has opened new possibilities. For instance, predictive modeling in data science uses algorithms to forecast outcomes based on historical data. This is particularly useful in fields like finance and marketing, where predicting consumer behavior can lead to better business strategies. The synergy between machine learning and statistics is creating innovative pathways, showcasing how statistical treatments are evolving in the age of big data and AI. This evolution is deeply tied to AI's value creation, which is shaped by its underlying philosophy.
Future Trends in Statistical Treatment for Research
Emerging Statistical Techniques
In recent years, new statistical methods are popping up that offer fresh ways to analyze data. These techniques are not just fancy add-ons; they actually help researchers handle complex data sets better. One emerging method is Bayesian statistics, which lets researchers update their findings as new data comes in, making the results more dynamic and relevant.
Impact of Big Data on Research
Big data is everywhere now, and it's changing how we do research. With so much information available, researchers can ask bigger questions and get more detailed answers. Big data allows for deeper insights into patterns and trends that were hard to see before. But with this comes the challenge of managing and interpreting vast amounts of data, which requires advanced tools and skills.
Integrating AI in Statistical Analysis
Artificial intelligence is becoming a big player in statistical analysis. AI can help automate complex calculations and uncover patterns that might be missed by human eyes. This integration is not just about speed; it's about accuracy and finding connections in data that were previously hidden. As AI continues to evolve, it promises to make statistical analysis more robust and insightful.
In conclusion, these trends are reshaping the landscape of statistical treatment in research. As we move forward, staying updated with these trends will be crucial for researchers aiming to leverage the full potential of their data.
As we look ahead, the future of statistical methods in research is bright and full of exciting possibilities. New technologies and approaches are changing how we analyze data, making it easier and more effective. If you're curious about how these trends can help you in your studies, visit our website for more information and resources that can guide you through your research journey!
Conclusion
In wrapping up our exploration of statistical methods, it's clear that understanding the basics can significantly enhance your research. You don't need to be a math whiz to grasp these concepts; a bit of patience and practice goes a long way. By breaking down complex data into manageable pieces, you can uncover insights that might otherwise remain hidden. Whether you're working on a thesis or a business report, these tools can help you make informed decisions. Remember, the key is to stay curious and keep questioning the numbers. With the right approach, statistics can be a powerful ally in your research journey.
Frequently Asked Questions
What is statistical treatment in research?
Statistical treatment refers to the methods used to analyze data in research. It helps researchers understand patterns, relationships, and trends in the data they collect.
Why is statistical treatment important in research?
Statistical treatment is crucial because it allows researchers to make sense of their data, draw conclusions, and support their findings with evidence. It helps in making informed decisions based on the data.
What are some common misconceptions about statistical treatment?
A common misconception is that statistical treatment is only for experts. In reality, basic statistical methods can be understood and applied by anyone with a little practice and the right tools.
How do I choose the right statistical method for my study?
Choosing the right method depends on your research question, the type of data you have, and the goals of your study. It's important to understand the strengths and limitations of different methods.
Can statistical treatment help in overcoming sample size limitations?
Yes, statistical methods can help address sample size issues by using techniques that maximize the information gained from a small sample. However, larger samples generally provide more reliable results.
What role does software play in statistical treatment?
Software can make statistical analysis easier by handling complex calculations and providing visualizations. It allows researchers to focus on interpreting results rather than crunching numbers manually.
Crunching the Numbers: Easy Statistical Treatments for Your Research

Ever stared at a pile of data and thought, "What now?" You're not alone. Research can be a maze, especially when numbers get involved. But here's the thing: statistical treatment isn't just for math whizzes. It's a tool that helps make sense of data, guiding you through the research labyrinth. In this article, we'll break down how statistical treatment in research works, why it's important, and how you can use it without losing your mind.
Key Takeaways
- Statistical treatment helps transform raw data into meaningful insights.
- Choosing the right statistical method is crucial for clear results.
- Balancing complexity with simplicity ensures your research is understandable.
- Reproducibility in statistical treatment boosts the credibility of your research.
- Understanding and interpreting statistical results is key to successful research.
Understanding the Role of Statistical Treatment in Research
Defining Statistical Treatment
Statistical treatment involves the application of statistical methods to collect, analyze, and interpret data. Imagine you're trying to figure out if a new teaching method is effective. You'd gather data from students who used the method and those who didn't, then apply statistical techniques to see if there's a significant difference in their performance. This process helps researchers make informed decisions based on data rather than guesswork.
Importance in Research Methodology
In research, statistical treatment is like the backbone that supports your entire study. Without it, your findings might just be random noise. It ensures that the results you get are reliable and not just a fluke. Think of it as using WhatsApp to communicate quickly and efficiently; statistical treatment helps convey the 'message' of your data clearly and accurately.
Common Misconceptions
Many people think statistical treatment is just about crunching numbers, but it's way more than that. It's about understanding what those numbers mean in the real world. Some folks believe it's only useful for large datasets, but even small studies can benefit from proper statistical analysis. Others might think it's too complicated, but with the right tools and guidance, anyone can grasp the basics.
Designing Effective Statistical Treatments for Research Studies
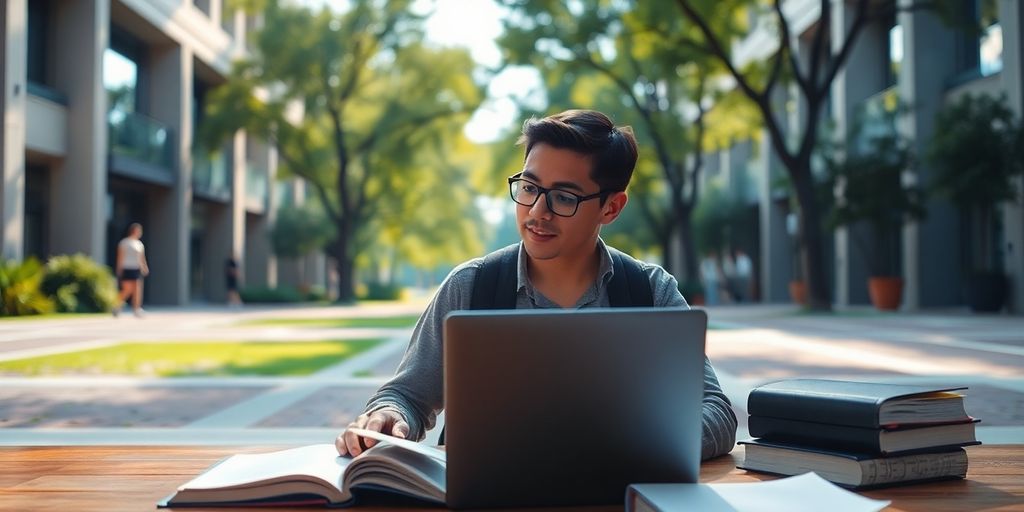
Choosing Appropriate Statistical Methods
Selecting the right statistical methods is like picking the right tool for a job. It's about understanding your research questions and the type of data you have. Choosing the wrong method can lead to misleading results. For instance, if you're analyzing survey data, you might consider logistic regression if your outcome is binary, or ANOVA if you're comparing means across groups. The choice should align with your study's goals, ensuring that the methods are robust enough to handle your data's complexity without oversimplifying the findings.
Balancing Complexity and Clarity
Research often walks a fine line between complexity and clarity. On one hand, sophisticated models can capture intricate data patterns, but they can also obscure the main findings if not presented clearly. It's crucial to balance these elements to make your research accessible. Here are a few tips:
- Simplify your language to explain complex models.
- Use visual aids like graphs and tables to illustrate key points.
- Focus on the main findings rather than getting lost in technical details.
Ensuring Reproducibility
In the world of research, reproducibility is king. It means others can follow your methods and arrive at the same results, which is essential for building trust and validating findings. To achieve this, document every step of your analysis process, from data collection to statistical testing. Use software tools that support reproducibility, such as R or Python, which allow you to share your code and data openly. This transparency not only strengthens your research but also aligns with practices advocated by platforms like LinkedIn, where professionals share insights and methodologies.
Incorporating these elements into your research design can not only enhance the quality of your study but also make the daunting task of "how to write your thesis in 30 days" a bit more manageable. By focusing on these core principles, you can ensure that your research is both rigorous and impactful.
Analyzing Data: Statistical Treatment Techniques
Descriptive vs. Inferential Statistics
When it comes to analyzing data, understanding the difference between descriptive and inferential statistics is key. Descriptive statistics provide a way to summarize and present data in a meaningful way, often using measures like mean, median, and mode. They help in giving a clear picture of what the data looks like. On the other hand, inferential statistics allow researchers to make predictions or inferences about a population based on a sample of data. This involves using data from a small group to make generalizations about a larger group. For example, if you survey 100 people about their favorite ice cream flavor, you might use inferential statistics to guess what the favorite flavor is for all people in a city.
Utilizing Software for Data Analysis
In today's digital age, using software for data analysis is almost a given. Programs like SPSS, R, and Python libraries such as Pandas and NumPy offer powerful tools for crunching numbers and visualizing data. These tools help in automating complex calculations, creating visualizations like graphs and charts, and even running simulations. They are essential for handling large datasets that would be impossible to analyze manually. The choice of software often depends on the complexity of the analysis and the user's familiarity with the tool.
Interpreting Statistical Results
Interpreting the results of statistical analysis is where the art meets the science. It's not just about understanding the numbers but also about what they mean in the context of your research. For instance, a p-value in a hypothesis test tells you whether your results are statistically significant. But it's equally important to consider the practical significance—does the result make a real-world difference? Misinterpretation can lead to incorrect conclusions, so it's crucial to carefully analyze and question the results. This guide on empirical analysis highlights the importance of considering context and variables to avoid misinterpretation.
Challenges in Applying Statistical Treatment in Research
Addressing Data Variability
When dealing with data, one of the biggest hurdles is its inherent variability. Data points can fluctuate, sometimes wildly, due to numerous factors like measurement errors or random sampling differences. This variability can obscure the true patterns researchers are trying to identify. For instance, a dataset gathered from Instagram might show trends that are more reflective of user engagement patterns rather than the actual phenomena being studied. Understanding and managing this variability is crucial to ensure that the statistical analysis remains robust and reliable.
Overcoming Sample Size Limitations
Sample size is another critical aspect that can significantly impact the outcomes of statistical treatments. A small sample size might not adequately represent the population, leading to skewed results. Researchers often face challenges in obtaining a large enough sample due to constraints like budget, time, or accessibility. To mitigate these issues, researchers might employ techniques like bootstrapping or use statistical software to simulate larger sample sizes, thereby enhancing the credibility of their findings.
Mitigating Bias and Errors
Bias and errors are persistent challenges in any research involving statistical treatment. Whether it's selection bias, where the sample isn't representative of the population, or measurement errors that skew data, these issues can lead to incorrect conclusions. Researchers must be diligent in designing their studies to minimize these biases. This might involve using random sampling methods, ensuring instruments are calibrated correctly, or employing blinding techniques in experimental designs. Acknowledging these biases and actively working to reduce them can significantly improve the quality of the research findings.
Enhancing Research Validity Through Statistical Treatment
Ensuring Statistical Significance
Statistical significance is like the backbone of research validity. It's about deciding if your findings are not just random flukes. Imagine you're flipping a coin, and it lands on heads ten times in a row. Is the coin rigged, or was that just luck? Statistical significance helps you figure that out. In research, a small p-value usually indicates that your results are statistically significant, meaning they're less likely to be due to chance. So, when you're conducting a study, it's crucial to set a threshold for this p-value to help determine the reliability of your findings.
Improving Confidence Intervals
Confidence intervals give you a range within which you can say, "I'm pretty sure the true value lies here." It's like saying, "I think the average height of students in this class is between 5'6" and 5'8"." This range helps to understand the precision of your estimate. A narrower confidence interval means more precision, while a wider one suggests more uncertainty. When you improve your confidence intervals, you're essentially tightening that range, which boosts the trustworthiness of your conclusions.
Generalizing Findings
Generalizing is about applying your study results to a broader population. Let's say you did a study on the effects of a new teaching method in one school. Can you say it'll work in all schools? Not necessarily. You need to ensure your sample is representative of the larger group you're interested in. This is where random sampling comes in handy. By choosing a sample that accurately reflects the population, you can make more confident generalizations. However, be cautious—differences in demographics or settings can affect how applicable your findings are elsewhere.
Incorporating these statistical treatments effectively can significantly enhance the validity of your research. They help ensure that your findings are not only accurate but also applicable to real-world scenarios, thus making your research more impactful.
Case Studies: Successful Statistical Treatments in Research
Healthcare Research Applications
In healthcare, statistical treatments are vital for interpreting complex data. Consider a study examining the impact of a new drug on blood pressure. Researchers might use a randomized control trial to assess the drug's effectiveness. By applying statistical methods like ANOVA (Analysis of Variance), they can determine whether changes in blood pressure are significant or just due to random variation. Such studies often reveal how statistical methods can uncover meaningful insights, ensuring that treatments are both effective and safe for patients.
Social Sciences Methodologies
In social sciences, statistical treatments help in understanding human behavior. For example, a study on the effects of educational interventions on student performance might employ regression analysis. This method helps in identifying the relationship between variables, such as teaching methods and student success. Regression analysis can highlight which interventions are truly beneficial, allowing educators to make informed decisions. This approach underscores the importance of statistical tools in developing effective educational strategies.
Innovations in Data Science
Data science has revolutionized how we approach research. In recent years, the integration of machine learning with statistical techniques has opened new possibilities. For instance, predictive modeling in data science uses algorithms to forecast outcomes based on historical data. This is particularly useful in fields like finance and marketing, where predicting consumer behavior can lead to better business strategies. The synergy between machine learning and statistics is creating innovative pathways, showcasing how statistical treatments are evolving in the age of big data and AI. This evolution is deeply tied to AI's value creation, which is shaped by its underlying philosophy.
Future Trends in Statistical Treatment for Research
Emerging Statistical Techniques
In recent years, new statistical methods are popping up that offer fresh ways to analyze data. These techniques are not just fancy add-ons; they actually help researchers handle complex data sets better. One emerging method is Bayesian statistics, which lets researchers update their findings as new data comes in, making the results more dynamic and relevant.
Impact of Big Data on Research
Big data is everywhere now, and it's changing how we do research. With so much information available, researchers can ask bigger questions and get more detailed answers. Big data allows for deeper insights into patterns and trends that were hard to see before. But with this comes the challenge of managing and interpreting vast amounts of data, which requires advanced tools and skills.
Integrating AI in Statistical Analysis
Artificial intelligence is becoming a big player in statistical analysis. AI can help automate complex calculations and uncover patterns that might be missed by human eyes. This integration is not just about speed; it's about accuracy and finding connections in data that were previously hidden. As AI continues to evolve, it promises to make statistical analysis more robust and insightful.
In conclusion, these trends are reshaping the landscape of statistical treatment in research. As we move forward, staying updated with these trends will be crucial for researchers aiming to leverage the full potential of their data.
As we look ahead, the future of statistical methods in research is bright and full of exciting possibilities. New technologies and approaches are changing how we analyze data, making it easier and more effective. If you're curious about how these trends can help you in your studies, visit our website for more information and resources that can guide you through your research journey!
Conclusion
In wrapping up our exploration of statistical methods, it's clear that understanding the basics can significantly enhance your research. You don't need to be a math whiz to grasp these concepts; a bit of patience and practice goes a long way. By breaking down complex data into manageable pieces, you can uncover insights that might otherwise remain hidden. Whether you're working on a thesis or a business report, these tools can help you make informed decisions. Remember, the key is to stay curious and keep questioning the numbers. With the right approach, statistics can be a powerful ally in your research journey.
Frequently Asked Questions
What is statistical treatment in research?
Statistical treatment refers to the methods used to analyze data in research. It helps researchers understand patterns, relationships, and trends in the data they collect.
Why is statistical treatment important in research?
Statistical treatment is crucial because it allows researchers to make sense of their data, draw conclusions, and support their findings with evidence. It helps in making informed decisions based on the data.
What are some common misconceptions about statistical treatment?
A common misconception is that statistical treatment is only for experts. In reality, basic statistical methods can be understood and applied by anyone with a little practice and the right tools.
How do I choose the right statistical method for my study?
Choosing the right method depends on your research question, the type of data you have, and the goals of your study. It's important to understand the strengths and limitations of different methods.
Can statistical treatment help in overcoming sample size limitations?
Yes, statistical methods can help address sample size issues by using techniques that maximize the information gained from a small sample. However, larger samples generally provide more reliable results.
What role does software play in statistical treatment?
Software can make statistical analysis easier by handling complex calculations and providing visualizations. It allows researchers to focus on interpreting results rather than crunching numbers manually.