Data Analysis Unlocked: Transform Your Data into Compelling Insights

Data analysis is like the secret sauce in research. It's what turns all those numbers and figures into something that actually makes sense. Whether you're diving into market research or trying to figure out why your latest experiment went sideways, data analysis is your go-to tool. It helps researchers uncover patterns, test theories, and make informed decisions. But let's be real—it's not always a walk in the park. There are challenges, like messy data and confusing results, but with the right approach, you can turn your raw data into insights that matter.
Key Takeaways
- Data analysis is crucial for turning raw data into meaningful insights.
- Choosing the right tools and methods is essential for effective analysis.
- Understanding both qualitative and quantitative data helps in comprehensive research.
- Data integrity and accuracy are vital for reliable results.
- Ethical practices in data analysis ensure privacy and unbiased results.
The Role of Data Analysis in Research
Understanding the Importance of Data Analysis
Data analysis is like the backbone of research. It helps researchers make sense of the numbers and patterns they see. Without it, research would just be a collection of random data points with no clear meaning. Data analysis turns raw numbers into meaningful insights, guiding decisions and helping researchers understand their findings more deeply. It's not just about crunching numbers; it's about finding the story behind the data.
How Data Analysis Enhances Research Outcomes
By using data analysis, researchers can improve the quality of their findings. It helps them see connections and patterns that might not be obvious at first. For example, in medical research, data analysis can identify trends in patient health, leading to better treatment plans. In environmental studies, it can show how changes in climate affect ecosystems. The ability to analyze data effectively can lead to more accurate and insightful conclusions.
Challenges in Data Analysis for Researchers
Data analysis isn't always easy. Researchers often face hurdles like messy data, which can lead to incorrect conclusions if not handled properly. There's also the challenge of choosing the right tools and methods for analysis. Plus, with the rise of big data, researchers need to manage and interpret vast amounts of information, which can be overwhelming. According to new research, there are nine critical factors that hinder organizations from making progress in data science, highlighting the complexity and challenges involved in effective data analysis.
Methodologies for Effective Data Analysis
Qualitative vs Quantitative Analysis
When it comes to analyzing data, choosing between qualitative and quantitative methods is a critical step. Qualitative analysis is all about understanding the non-numerical aspects of data, like themes, patterns, and narratives. It’s often used in social sciences to explore complex human behaviors and societal trends. On the other hand, quantitative analysis focuses on numbers and statistical models. It’s great for testing hypotheses and making predictions based on numerical data. The choice between these two depends largely on the research question and the nature of the data you have.
Choosing the Right Analytical Tools
In the world of data analysis, picking the right tools can make a huge difference. There’s a wide range of software available, from basic spreadsheet programs to advanced statistical software like SPSS or machine learning tools. For those dealing with large datasets, platforms like Tableau or Power BI are excellent for visualizing data. Meanwhile, programming languages like Python and R offer flexibility and power in handling complex data sets. Selecting the right tool is not just about the software’s capabilities but also about how well it fits the specific needs of your project.
Ensuring Data Integrity and Accuracy
Data integrity and accuracy are vital for any analysis. Mistakes in data can lead to incorrect conclusions, so it’s important to ensure your data is clean and reliable. This involves checking for errors, handling missing values, and verifying the data sources. A good practice is to maintain a data audit trail, which documents all changes and updates made to the dataset. This not only helps in maintaining accuracy but also in making the analysis process transparent and reproducible. Keeping your data in check ensures that your findings are trustworthy and actionable.
Transforming Raw Data into Actionable Insights
Steps to Clean and Prepare Data
Cleaning and preparing data is like tidying up a messy room. It’s essential before you can make sense of anything. Start by sorting through your data to remove any duplicates or errors. Then, standardize your data formats so everything lines up nicely. This might mean converting dates into the same format or ensuring all text fields use the same case. Finally, fill in any missing data where possible, or decide if those gaps are too big to ignore.
Techniques for Data Interpretation
Once your data is clean, it’s time to figure out what it’s telling you. This step is all about looking for patterns or trends. You might use statistical methods or software to analyze your data. Think of it like putting together a puzzle—sometimes you need to try different pieces to see what fits. Visualization tools can be a huge help here, turning complex numbers into charts or graphs that are easier to understand.
Leveraging Insights for Strategic Decisions
Now that you’ve interpreted your data, it’s time to put those insights to work. This is where you can really make a difference. Use what you’ve learned to inform your strategies and decisions. Maybe you’ve found a new market opportunity or identified a process that needs improvement. It’s about turning your findings into actions that align with your goals. Remember, insights are only useful if they lead to action. For example, managers should prioritize actions based on impact and feasibility, creating structured plans and monitoring outcomes for improvement. Embracing a data-driven culture, like what’s practiced on LinkedIn, can enhance the effectiveness of these insights.
By following these steps, you can transform raw data into insights that not only support strategic decisions but also drive meaningful outcomes. The key is to ensure that every piece of data is aligned with your organizational goals, making it a powerful tool for success.
Advanced Tools and Techniques in Data Analysis
Utilizing Machine Learning Algorithms
Machine learning is reshaping how we analyze data, making it possible to uncover patterns that weren't visible before. Algorithms like decision trees, neural networks, and support vector machines are some of the tools that analysts use to predict outcomes and classify data. Machine learning's ability to adapt and learn from new data makes it a powerful tool in data analysis. It helps in identifying trends and making forecasts, which are crucial for strategic planning.
Data Visualization for Enhanced Understanding
Turning data into visuals can make complex information more accessible. Tools like Tableau and Power BI allow analysts to create interactive dashboards and charts, helping stakeholders to quickly grasp data insights. The key is to choose the right type of visualization—whether it's a pie chart, line graph, or scatter plot—depending on the data and the message you want to convey. A well-crafted visual can often communicate insights more effectively than raw numbers.
The Role of Statistical Software in Analysis
Statistical software like SPSS and SAS are essential for handling large datasets and performing complex analyses. These tools offer a range of functionalities, from basic descriptive statistics to advanced regression analysis and clustering. They help in organizing data, running statistical tests, and interpreting results. Using these tools, analysts can derive meaningful insights that inform decision-making processes.
Ethical Considerations in Data Analysis
Ensuring Data Privacy and Security
In today's data-driven world, keeping data private and secure is a big deal. With so much data floating around, especially on platforms like WhatsApp, there are risks. Data breaches can happen, leading to sensitive information being exposed. So, it's super important to have strong security measures in place. This includes encryption, access controls, and regular audits to ensure that data is safe from unauthorized access.
Addressing Bias in Data Interpretation
Bias in data analysis is a tricky issue. It can creep in during data collection, analysis, or even in the way results are interpreted. If not handled carefully, bias can lead to wrong conclusions. Analysts need to be aware of their own biases and work to minimize them. This means using diverse data sources and employing techniques like random sampling and blind studies to get a clearer picture.
The Impact of Ethical Practices on Research
Ethical practices in data analysis are not just about following rules. They're about maintaining trust and credibility in research. When researchers adhere to ethical standards, it enhances the reliability of their findings. This, in turn, builds trust with stakeholders and the public. Ethical practices also help in avoiding legal issues, which can arise from unethical data handling.
Future Trends in Data Analysis for Research
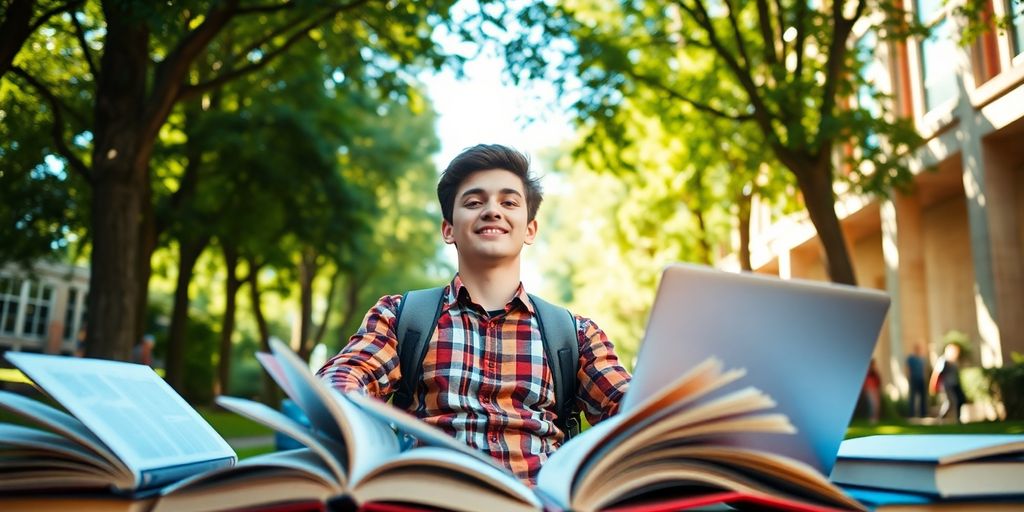
The Rise of Big Data and Its Implications
Big data is not just a buzzword anymore; it's a reality that's reshaping research. With massive amounts of information generated every second, researchers have an unprecedented opportunity to uncover patterns and insights that were previously hidden. However, handling such vast datasets comes with its own set of challenges, like ensuring data quality and managing storage. As we move forward, tools that can efficiently process and analyze big data will become indispensable. Researchers need to adapt to these changes to stay relevant.
Integration of AI in Data Analysis
Artificial Intelligence (AI) is making waves in how data is analyzed. AI algorithms can sift through large datasets quickly, identifying trends and making predictions that would take humans much longer to uncover. This trend in AI is not only speeding up the research process but also increasing its accuracy. Expect to see more AI-driven tools that assist researchers in everything from data collection to interpretation.
Predictive Analytics in Research
Predictive analytics is all about using historical data to predict future outcomes. In research, this means being able to foresee trends and make informed decisions ahead of time. This approach is particularly useful in fields like healthcare, where predicting patient outcomes can significantly improve treatment plans. As predictive analytics becomes more sophisticated, its role in research will only grow, offering insights that were once thought impossible.
In summary, the future of data analysis in research is bright and full of potential. By embracing big data, AI, and predictive analytics, researchers can unlock insights that drive innovation and progress in their fields.
Building a Data-Driven Research Culture
Fostering Collaboration Among Researchers
Creating a data-driven culture isn't just about having the right tools; it's about building an environment where collaboration thrives. Researchers need to share insights and methodologies openly to push the boundaries of what's possible. This can be achieved through regular workshops, collaborative platforms, and peer reviews that encourage sharing of data and findings. Using platforms like Instagram for sharing quick insights and updates can also keep the team engaged and informed.
Training and Development in Data Skills
For a research culture to truly embrace data-driven approaches, ongoing training is essential. This means offering workshops and courses that focus on the latest data analysis techniques and tools. From learning how to write your thesis in 30 days to mastering complex data visualization tools, researchers should always be encouraged to expand their skill sets. Providing access to online courses and certifications can keep the team updated with the latest trends and methodologies.
Creating a Supportive Environment for Data Innovation
Innovation thrives in a supportive environment. This involves not just having access to the latest tools and technologies, but also fostering a mindset that encourages experimentation and risk-taking. Encourage researchers to explore new data analysis methods and to share their findings openly. Regular brainstorming sessions and innovation hubs can be effective in nurturing new ideas. Providing a structured yet flexible framework can help researchers feel supported as they explore new avenues in data-driven research.
Creating a research culture that relies on data can really change how we learn and grow. If you're feeling lost or stressed about your thesis, don't worry! We have a simple plan to help you through it. Visit our website to discover how our Thesis Action Plan can guide you step by step. Take the first step towards a smoother thesis journey today!
Conclusion
In wrapping up our exploration of data analysis, it's clear that turning raw numbers into meaningful insights is more than just a technical skill—it's an art form. As we've seen, the process involves not only collecting and cleaning data but also interpreting it in ways that can drive real-world decisions. Whether you're a seasoned analyst or just starting out, the key is to remain curious and open to learning. The tools and techniques available today offer endless possibilities for those willing to dive in and explore. Remember, the goal is not just to crunch numbers but to tell a story with your data, one that can inform and inspire. So, keep experimenting, keep questioning, and most importantly, keep analyzing. The insights you uncover could be the key to unlocking new opportunities and driving success in your field.
Frequently Asked Questions
What is data analysis?
Data analysis is the process of examining raw data to find patterns, draw conclusions, and make decisions. It's like solving a puzzle with numbers and facts.
Why is data analysis important for research?
Data analysis helps researchers understand their data better, find patterns, and make informed decisions. It turns numbers into useful information.
What are some common challenges in data analysis?
Some common challenges include dealing with messy data, choosing the right tools, and making sure the analysis is accurate and unbiased.
How can I ensure data accuracy in my analysis?
To ensure data accuracy, double-check your data sources, clean your data to remove errors, and use reliable tools and methods for analysis.
What tools are used in data analysis?
Common tools include spreadsheets like Excel, programming languages like Python, and specialized software like Tableau for data visualization.
What is the difference between qualitative and quantitative analysis?
Qualitative analysis looks at non-numerical data like opinions and feelings, while quantitative analysis deals with numbers and statistics.
Data Analysis Unlocked: Transform Your Data into Compelling Insights

Data analysis is like the secret sauce in research. It's what turns all those numbers and figures into something that actually makes sense. Whether you're diving into market research or trying to figure out why your latest experiment went sideways, data analysis is your go-to tool. It helps researchers uncover patterns, test theories, and make informed decisions. But let's be real—it's not always a walk in the park. There are challenges, like messy data and confusing results, but with the right approach, you can turn your raw data into insights that matter.
Key Takeaways
- Data analysis is crucial for turning raw data into meaningful insights.
- Choosing the right tools and methods is essential for effective analysis.
- Understanding both qualitative and quantitative data helps in comprehensive research.
- Data integrity and accuracy are vital for reliable results.
- Ethical practices in data analysis ensure privacy and unbiased results.
The Role of Data Analysis in Research
Understanding the Importance of Data Analysis
Data analysis is like the backbone of research. It helps researchers make sense of the numbers and patterns they see. Without it, research would just be a collection of random data points with no clear meaning. Data analysis turns raw numbers into meaningful insights, guiding decisions and helping researchers understand their findings more deeply. It's not just about crunching numbers; it's about finding the story behind the data.
How Data Analysis Enhances Research Outcomes
By using data analysis, researchers can improve the quality of their findings. It helps them see connections and patterns that might not be obvious at first. For example, in medical research, data analysis can identify trends in patient health, leading to better treatment plans. In environmental studies, it can show how changes in climate affect ecosystems. The ability to analyze data effectively can lead to more accurate and insightful conclusions.
Challenges in Data Analysis for Researchers
Data analysis isn't always easy. Researchers often face hurdles like messy data, which can lead to incorrect conclusions if not handled properly. There's also the challenge of choosing the right tools and methods for analysis. Plus, with the rise of big data, researchers need to manage and interpret vast amounts of information, which can be overwhelming. According to new research, there are nine critical factors that hinder organizations from making progress in data science, highlighting the complexity and challenges involved in effective data analysis.
Methodologies for Effective Data Analysis
Qualitative vs Quantitative Analysis
When it comes to analyzing data, choosing between qualitative and quantitative methods is a critical step. Qualitative analysis is all about understanding the non-numerical aspects of data, like themes, patterns, and narratives. It’s often used in social sciences to explore complex human behaviors and societal trends. On the other hand, quantitative analysis focuses on numbers and statistical models. It’s great for testing hypotheses and making predictions based on numerical data. The choice between these two depends largely on the research question and the nature of the data you have.
Choosing the Right Analytical Tools
In the world of data analysis, picking the right tools can make a huge difference. There’s a wide range of software available, from basic spreadsheet programs to advanced statistical software like SPSS or machine learning tools. For those dealing with large datasets, platforms like Tableau or Power BI are excellent for visualizing data. Meanwhile, programming languages like Python and R offer flexibility and power in handling complex data sets. Selecting the right tool is not just about the software’s capabilities but also about how well it fits the specific needs of your project.
Ensuring Data Integrity and Accuracy
Data integrity and accuracy are vital for any analysis. Mistakes in data can lead to incorrect conclusions, so it’s important to ensure your data is clean and reliable. This involves checking for errors, handling missing values, and verifying the data sources. A good practice is to maintain a data audit trail, which documents all changes and updates made to the dataset. This not only helps in maintaining accuracy but also in making the analysis process transparent and reproducible. Keeping your data in check ensures that your findings are trustworthy and actionable.
Transforming Raw Data into Actionable Insights
Steps to Clean and Prepare Data
Cleaning and preparing data is like tidying up a messy room. It’s essential before you can make sense of anything. Start by sorting through your data to remove any duplicates or errors. Then, standardize your data formats so everything lines up nicely. This might mean converting dates into the same format or ensuring all text fields use the same case. Finally, fill in any missing data where possible, or decide if those gaps are too big to ignore.
Techniques for Data Interpretation
Once your data is clean, it’s time to figure out what it’s telling you. This step is all about looking for patterns or trends. You might use statistical methods or software to analyze your data. Think of it like putting together a puzzle—sometimes you need to try different pieces to see what fits. Visualization tools can be a huge help here, turning complex numbers into charts or graphs that are easier to understand.
Leveraging Insights for Strategic Decisions
Now that you’ve interpreted your data, it’s time to put those insights to work. This is where you can really make a difference. Use what you’ve learned to inform your strategies and decisions. Maybe you’ve found a new market opportunity or identified a process that needs improvement. It’s about turning your findings into actions that align with your goals. Remember, insights are only useful if they lead to action. For example, managers should prioritize actions based on impact and feasibility, creating structured plans and monitoring outcomes for improvement. Embracing a data-driven culture, like what’s practiced on LinkedIn, can enhance the effectiveness of these insights.
By following these steps, you can transform raw data into insights that not only support strategic decisions but also drive meaningful outcomes. The key is to ensure that every piece of data is aligned with your organizational goals, making it a powerful tool for success.
Advanced Tools and Techniques in Data Analysis
Utilizing Machine Learning Algorithms
Machine learning is reshaping how we analyze data, making it possible to uncover patterns that weren't visible before. Algorithms like decision trees, neural networks, and support vector machines are some of the tools that analysts use to predict outcomes and classify data. Machine learning's ability to adapt and learn from new data makes it a powerful tool in data analysis. It helps in identifying trends and making forecasts, which are crucial for strategic planning.
Data Visualization for Enhanced Understanding
Turning data into visuals can make complex information more accessible. Tools like Tableau and Power BI allow analysts to create interactive dashboards and charts, helping stakeholders to quickly grasp data insights. The key is to choose the right type of visualization—whether it's a pie chart, line graph, or scatter plot—depending on the data and the message you want to convey. A well-crafted visual can often communicate insights more effectively than raw numbers.
The Role of Statistical Software in Analysis
Statistical software like SPSS and SAS are essential for handling large datasets and performing complex analyses. These tools offer a range of functionalities, from basic descriptive statistics to advanced regression analysis and clustering. They help in organizing data, running statistical tests, and interpreting results. Using these tools, analysts can derive meaningful insights that inform decision-making processes.
Ethical Considerations in Data Analysis
Ensuring Data Privacy and Security
In today's data-driven world, keeping data private and secure is a big deal. With so much data floating around, especially on platforms like WhatsApp, there are risks. Data breaches can happen, leading to sensitive information being exposed. So, it's super important to have strong security measures in place. This includes encryption, access controls, and regular audits to ensure that data is safe from unauthorized access.
Addressing Bias in Data Interpretation
Bias in data analysis is a tricky issue. It can creep in during data collection, analysis, or even in the way results are interpreted. If not handled carefully, bias can lead to wrong conclusions. Analysts need to be aware of their own biases and work to minimize them. This means using diverse data sources and employing techniques like random sampling and blind studies to get a clearer picture.
The Impact of Ethical Practices on Research
Ethical practices in data analysis are not just about following rules. They're about maintaining trust and credibility in research. When researchers adhere to ethical standards, it enhances the reliability of their findings. This, in turn, builds trust with stakeholders and the public. Ethical practices also help in avoiding legal issues, which can arise from unethical data handling.
Future Trends in Data Analysis for Research
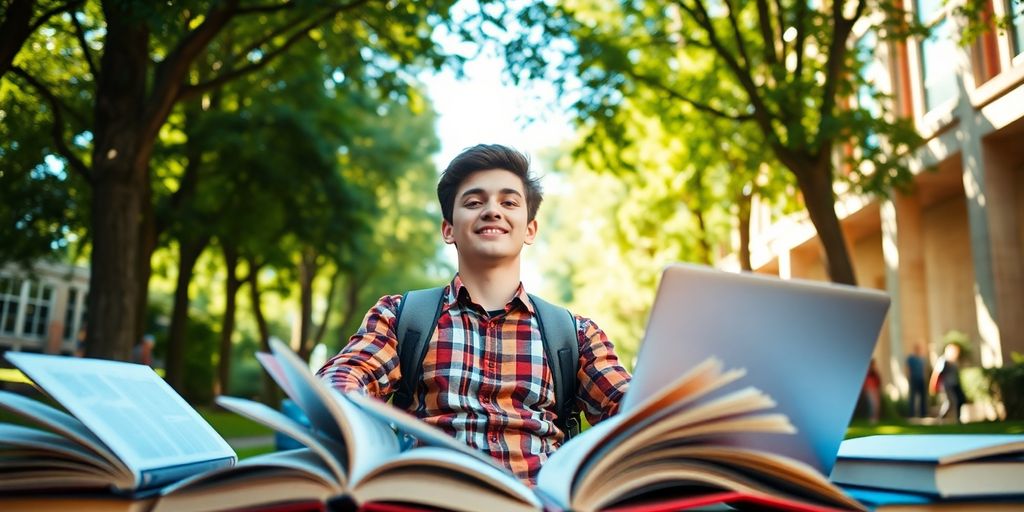
The Rise of Big Data and Its Implications
Big data is not just a buzzword anymore; it's a reality that's reshaping research. With massive amounts of information generated every second, researchers have an unprecedented opportunity to uncover patterns and insights that were previously hidden. However, handling such vast datasets comes with its own set of challenges, like ensuring data quality and managing storage. As we move forward, tools that can efficiently process and analyze big data will become indispensable. Researchers need to adapt to these changes to stay relevant.
Integration of AI in Data Analysis
Artificial Intelligence (AI) is making waves in how data is analyzed. AI algorithms can sift through large datasets quickly, identifying trends and making predictions that would take humans much longer to uncover. This trend in AI is not only speeding up the research process but also increasing its accuracy. Expect to see more AI-driven tools that assist researchers in everything from data collection to interpretation.
Predictive Analytics in Research
Predictive analytics is all about using historical data to predict future outcomes. In research, this means being able to foresee trends and make informed decisions ahead of time. This approach is particularly useful in fields like healthcare, where predicting patient outcomes can significantly improve treatment plans. As predictive analytics becomes more sophisticated, its role in research will only grow, offering insights that were once thought impossible.
In summary, the future of data analysis in research is bright and full of potential. By embracing big data, AI, and predictive analytics, researchers can unlock insights that drive innovation and progress in their fields.
Building a Data-Driven Research Culture
Fostering Collaboration Among Researchers
Creating a data-driven culture isn't just about having the right tools; it's about building an environment where collaboration thrives. Researchers need to share insights and methodologies openly to push the boundaries of what's possible. This can be achieved through regular workshops, collaborative platforms, and peer reviews that encourage sharing of data and findings. Using platforms like Instagram for sharing quick insights and updates can also keep the team engaged and informed.
Training and Development in Data Skills
For a research culture to truly embrace data-driven approaches, ongoing training is essential. This means offering workshops and courses that focus on the latest data analysis techniques and tools. From learning how to write your thesis in 30 days to mastering complex data visualization tools, researchers should always be encouraged to expand their skill sets. Providing access to online courses and certifications can keep the team updated with the latest trends and methodologies.
Creating a Supportive Environment for Data Innovation
Innovation thrives in a supportive environment. This involves not just having access to the latest tools and technologies, but also fostering a mindset that encourages experimentation and risk-taking. Encourage researchers to explore new data analysis methods and to share their findings openly. Regular brainstorming sessions and innovation hubs can be effective in nurturing new ideas. Providing a structured yet flexible framework can help researchers feel supported as they explore new avenues in data-driven research.
Creating a research culture that relies on data can really change how we learn and grow. If you're feeling lost or stressed about your thesis, don't worry! We have a simple plan to help you through it. Visit our website to discover how our Thesis Action Plan can guide you step by step. Take the first step towards a smoother thesis journey today!
Conclusion
In wrapping up our exploration of data analysis, it's clear that turning raw numbers into meaningful insights is more than just a technical skill—it's an art form. As we've seen, the process involves not only collecting and cleaning data but also interpreting it in ways that can drive real-world decisions. Whether you're a seasoned analyst or just starting out, the key is to remain curious and open to learning. The tools and techniques available today offer endless possibilities for those willing to dive in and explore. Remember, the goal is not just to crunch numbers but to tell a story with your data, one that can inform and inspire. So, keep experimenting, keep questioning, and most importantly, keep analyzing. The insights you uncover could be the key to unlocking new opportunities and driving success in your field.
Frequently Asked Questions
What is data analysis?
Data analysis is the process of examining raw data to find patterns, draw conclusions, and make decisions. It's like solving a puzzle with numbers and facts.
Why is data analysis important for research?
Data analysis helps researchers understand their data better, find patterns, and make informed decisions. It turns numbers into useful information.
What are some common challenges in data analysis?
Some common challenges include dealing with messy data, choosing the right tools, and making sure the analysis is accurate and unbiased.
How can I ensure data accuracy in my analysis?
To ensure data accuracy, double-check your data sources, clean your data to remove errors, and use reliable tools and methods for analysis.
What tools are used in data analysis?
Common tools include spreadsheets like Excel, programming languages like Python, and specialized software like Tableau for data visualization.
What is the difference between qualitative and quantitative analysis?
Qualitative analysis looks at non-numerical data like opinions and feelings, while quantitative analysis deals with numbers and statistics.
Data Analysis Unlocked: Transform Your Data into Compelling Insights

Data analysis is like the secret sauce in research. It's what turns all those numbers and figures into something that actually makes sense. Whether you're diving into market research or trying to figure out why your latest experiment went sideways, data analysis is your go-to tool. It helps researchers uncover patterns, test theories, and make informed decisions. But let's be real—it's not always a walk in the park. There are challenges, like messy data and confusing results, but with the right approach, you can turn your raw data into insights that matter.
Key Takeaways
- Data analysis is crucial for turning raw data into meaningful insights.
- Choosing the right tools and methods is essential for effective analysis.
- Understanding both qualitative and quantitative data helps in comprehensive research.
- Data integrity and accuracy are vital for reliable results.
- Ethical practices in data analysis ensure privacy and unbiased results.
The Role of Data Analysis in Research
Understanding the Importance of Data Analysis
Data analysis is like the backbone of research. It helps researchers make sense of the numbers and patterns they see. Without it, research would just be a collection of random data points with no clear meaning. Data analysis turns raw numbers into meaningful insights, guiding decisions and helping researchers understand their findings more deeply. It's not just about crunching numbers; it's about finding the story behind the data.
How Data Analysis Enhances Research Outcomes
By using data analysis, researchers can improve the quality of their findings. It helps them see connections and patterns that might not be obvious at first. For example, in medical research, data analysis can identify trends in patient health, leading to better treatment plans. In environmental studies, it can show how changes in climate affect ecosystems. The ability to analyze data effectively can lead to more accurate and insightful conclusions.
Challenges in Data Analysis for Researchers
Data analysis isn't always easy. Researchers often face hurdles like messy data, which can lead to incorrect conclusions if not handled properly. There's also the challenge of choosing the right tools and methods for analysis. Plus, with the rise of big data, researchers need to manage and interpret vast amounts of information, which can be overwhelming. According to new research, there are nine critical factors that hinder organizations from making progress in data science, highlighting the complexity and challenges involved in effective data analysis.
Methodologies for Effective Data Analysis
Qualitative vs Quantitative Analysis
When it comes to analyzing data, choosing between qualitative and quantitative methods is a critical step. Qualitative analysis is all about understanding the non-numerical aspects of data, like themes, patterns, and narratives. It’s often used in social sciences to explore complex human behaviors and societal trends. On the other hand, quantitative analysis focuses on numbers and statistical models. It’s great for testing hypotheses and making predictions based on numerical data. The choice between these two depends largely on the research question and the nature of the data you have.
Choosing the Right Analytical Tools
In the world of data analysis, picking the right tools can make a huge difference. There’s a wide range of software available, from basic spreadsheet programs to advanced statistical software like SPSS or machine learning tools. For those dealing with large datasets, platforms like Tableau or Power BI are excellent for visualizing data. Meanwhile, programming languages like Python and R offer flexibility and power in handling complex data sets. Selecting the right tool is not just about the software’s capabilities but also about how well it fits the specific needs of your project.
Ensuring Data Integrity and Accuracy
Data integrity and accuracy are vital for any analysis. Mistakes in data can lead to incorrect conclusions, so it’s important to ensure your data is clean and reliable. This involves checking for errors, handling missing values, and verifying the data sources. A good practice is to maintain a data audit trail, which documents all changes and updates made to the dataset. This not only helps in maintaining accuracy but also in making the analysis process transparent and reproducible. Keeping your data in check ensures that your findings are trustworthy and actionable.
Transforming Raw Data into Actionable Insights
Steps to Clean and Prepare Data
Cleaning and preparing data is like tidying up a messy room. It’s essential before you can make sense of anything. Start by sorting through your data to remove any duplicates or errors. Then, standardize your data formats so everything lines up nicely. This might mean converting dates into the same format or ensuring all text fields use the same case. Finally, fill in any missing data where possible, or decide if those gaps are too big to ignore.
Techniques for Data Interpretation
Once your data is clean, it’s time to figure out what it’s telling you. This step is all about looking for patterns or trends. You might use statistical methods or software to analyze your data. Think of it like putting together a puzzle—sometimes you need to try different pieces to see what fits. Visualization tools can be a huge help here, turning complex numbers into charts or graphs that are easier to understand.
Leveraging Insights for Strategic Decisions
Now that you’ve interpreted your data, it’s time to put those insights to work. This is where you can really make a difference. Use what you’ve learned to inform your strategies and decisions. Maybe you’ve found a new market opportunity or identified a process that needs improvement. It’s about turning your findings into actions that align with your goals. Remember, insights are only useful if they lead to action. For example, managers should prioritize actions based on impact and feasibility, creating structured plans and monitoring outcomes for improvement. Embracing a data-driven culture, like what’s practiced on LinkedIn, can enhance the effectiveness of these insights.
By following these steps, you can transform raw data into insights that not only support strategic decisions but also drive meaningful outcomes. The key is to ensure that every piece of data is aligned with your organizational goals, making it a powerful tool for success.
Advanced Tools and Techniques in Data Analysis
Utilizing Machine Learning Algorithms
Machine learning is reshaping how we analyze data, making it possible to uncover patterns that weren't visible before. Algorithms like decision trees, neural networks, and support vector machines are some of the tools that analysts use to predict outcomes and classify data. Machine learning's ability to adapt and learn from new data makes it a powerful tool in data analysis. It helps in identifying trends and making forecasts, which are crucial for strategic planning.
Data Visualization for Enhanced Understanding
Turning data into visuals can make complex information more accessible. Tools like Tableau and Power BI allow analysts to create interactive dashboards and charts, helping stakeholders to quickly grasp data insights. The key is to choose the right type of visualization—whether it's a pie chart, line graph, or scatter plot—depending on the data and the message you want to convey. A well-crafted visual can often communicate insights more effectively than raw numbers.
The Role of Statistical Software in Analysis
Statistical software like SPSS and SAS are essential for handling large datasets and performing complex analyses. These tools offer a range of functionalities, from basic descriptive statistics to advanced regression analysis and clustering. They help in organizing data, running statistical tests, and interpreting results. Using these tools, analysts can derive meaningful insights that inform decision-making processes.
Ethical Considerations in Data Analysis
Ensuring Data Privacy and Security
In today's data-driven world, keeping data private and secure is a big deal. With so much data floating around, especially on platforms like WhatsApp, there are risks. Data breaches can happen, leading to sensitive information being exposed. So, it's super important to have strong security measures in place. This includes encryption, access controls, and regular audits to ensure that data is safe from unauthorized access.
Addressing Bias in Data Interpretation
Bias in data analysis is a tricky issue. It can creep in during data collection, analysis, or even in the way results are interpreted. If not handled carefully, bias can lead to wrong conclusions. Analysts need to be aware of their own biases and work to minimize them. This means using diverse data sources and employing techniques like random sampling and blind studies to get a clearer picture.
The Impact of Ethical Practices on Research
Ethical practices in data analysis are not just about following rules. They're about maintaining trust and credibility in research. When researchers adhere to ethical standards, it enhances the reliability of their findings. This, in turn, builds trust with stakeholders and the public. Ethical practices also help in avoiding legal issues, which can arise from unethical data handling.
Future Trends in Data Analysis for Research
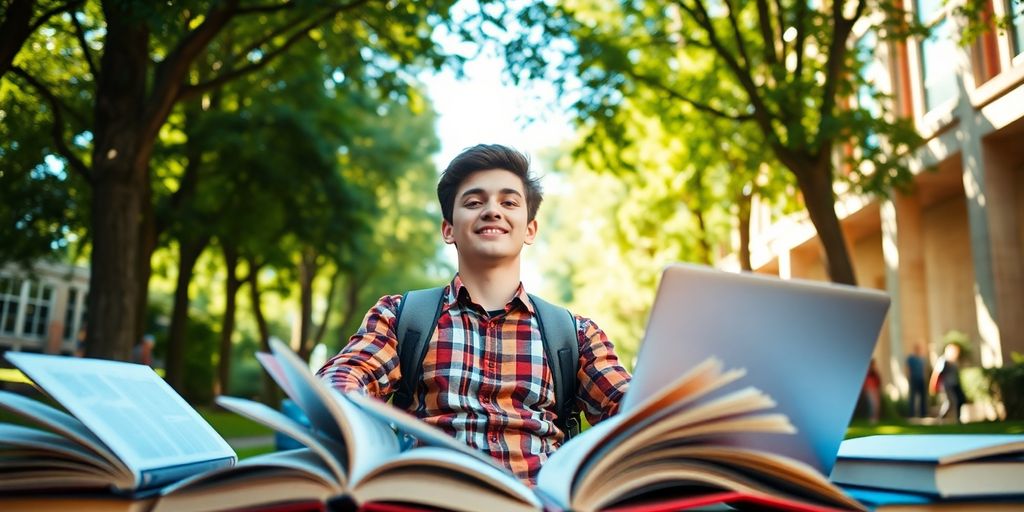
The Rise of Big Data and Its Implications
Big data is not just a buzzword anymore; it's a reality that's reshaping research. With massive amounts of information generated every second, researchers have an unprecedented opportunity to uncover patterns and insights that were previously hidden. However, handling such vast datasets comes with its own set of challenges, like ensuring data quality and managing storage. As we move forward, tools that can efficiently process and analyze big data will become indispensable. Researchers need to adapt to these changes to stay relevant.
Integration of AI in Data Analysis
Artificial Intelligence (AI) is making waves in how data is analyzed. AI algorithms can sift through large datasets quickly, identifying trends and making predictions that would take humans much longer to uncover. This trend in AI is not only speeding up the research process but also increasing its accuracy. Expect to see more AI-driven tools that assist researchers in everything from data collection to interpretation.
Predictive Analytics in Research
Predictive analytics is all about using historical data to predict future outcomes. In research, this means being able to foresee trends and make informed decisions ahead of time. This approach is particularly useful in fields like healthcare, where predicting patient outcomes can significantly improve treatment plans. As predictive analytics becomes more sophisticated, its role in research will only grow, offering insights that were once thought impossible.
In summary, the future of data analysis in research is bright and full of potential. By embracing big data, AI, and predictive analytics, researchers can unlock insights that drive innovation and progress in their fields.
Building a Data-Driven Research Culture
Fostering Collaboration Among Researchers
Creating a data-driven culture isn't just about having the right tools; it's about building an environment where collaboration thrives. Researchers need to share insights and methodologies openly to push the boundaries of what's possible. This can be achieved through regular workshops, collaborative platforms, and peer reviews that encourage sharing of data and findings. Using platforms like Instagram for sharing quick insights and updates can also keep the team engaged and informed.
Training and Development in Data Skills
For a research culture to truly embrace data-driven approaches, ongoing training is essential. This means offering workshops and courses that focus on the latest data analysis techniques and tools. From learning how to write your thesis in 30 days to mastering complex data visualization tools, researchers should always be encouraged to expand their skill sets. Providing access to online courses and certifications can keep the team updated with the latest trends and methodologies.
Creating a Supportive Environment for Data Innovation
Innovation thrives in a supportive environment. This involves not just having access to the latest tools and technologies, but also fostering a mindset that encourages experimentation and risk-taking. Encourage researchers to explore new data analysis methods and to share their findings openly. Regular brainstorming sessions and innovation hubs can be effective in nurturing new ideas. Providing a structured yet flexible framework can help researchers feel supported as they explore new avenues in data-driven research.
Creating a research culture that relies on data can really change how we learn and grow. If you're feeling lost or stressed about your thesis, don't worry! We have a simple plan to help you through it. Visit our website to discover how our Thesis Action Plan can guide you step by step. Take the first step towards a smoother thesis journey today!
Conclusion
In wrapping up our exploration of data analysis, it's clear that turning raw numbers into meaningful insights is more than just a technical skill—it's an art form. As we've seen, the process involves not only collecting and cleaning data but also interpreting it in ways that can drive real-world decisions. Whether you're a seasoned analyst or just starting out, the key is to remain curious and open to learning. The tools and techniques available today offer endless possibilities for those willing to dive in and explore. Remember, the goal is not just to crunch numbers but to tell a story with your data, one that can inform and inspire. So, keep experimenting, keep questioning, and most importantly, keep analyzing. The insights you uncover could be the key to unlocking new opportunities and driving success in your field.
Frequently Asked Questions
What is data analysis?
Data analysis is the process of examining raw data to find patterns, draw conclusions, and make decisions. It's like solving a puzzle with numbers and facts.
Why is data analysis important for research?
Data analysis helps researchers understand their data better, find patterns, and make informed decisions. It turns numbers into useful information.
What are some common challenges in data analysis?
Some common challenges include dealing with messy data, choosing the right tools, and making sure the analysis is accurate and unbiased.
How can I ensure data accuracy in my analysis?
To ensure data accuracy, double-check your data sources, clean your data to remove errors, and use reliable tools and methods for analysis.
What tools are used in data analysis?
Common tools include spreadsheets like Excel, programming languages like Python, and specialized software like Tableau for data visualization.
What is the difference between qualitative and quantitative analysis?
Qualitative analysis looks at non-numerical data like opinions and feelings, while quantitative analysis deals with numbers and statistics.