Experimental Design Breakdown: Which Type Fits Your Study?

Ever found yourself tangled in the web of experimental design? You're not alone. It's like trying to choose the right tool from a massive toolbox without knowing what each does. Experimental design, at its core, is about structuring your research so you can get clear, reliable results. But with so many types out there, it can feel overwhelming. This article is here to break down the different types of experimental design and help you figure out which one suits your study best.
Key Takeaways
- Experimental design is essential for reliable research outcomes.
- Different designs suit different study needs and questions.
- Completely randomized designs are straightforward but may lack control over variables.
- Randomized block designs help account for variability within groups.
- Factorial designs allow for the study of multiple factors simultaneously.
Understanding the Foundations of Experimental Design
Defining Experimental Design
Experimental design is the blueprint for conducting research that seeks to establish cause-and-effect relationships. It involves the careful planning of how to manipulate one or more independent variables to observe their effects on dependent variables. This structured approach ensures that the results are reliable and can be attributed to the variables being tested, rather than external factors.
Importance of Experimental Design in Research
The significance of experimental design in research cannot be overstated. A well-structured experimental design enhances the credibility of a study by minimizing biases and errors. It provides a framework for testing hypotheses in a controlled environment, thereby yielding results that are both replicable and generalizable. For researchers, especially those sharing their findings on platforms like LinkedIn, a robust experimental design is essential for gaining trust and recognition in the academic community.
Key Components of Experimental Design
An effective experimental design comprises several key components:
- Variables: These include independent variables (manipulated by the researcher) and dependent variables (measured outcomes).
- Control Groups: Used to compare against experimental groups to ascertain the effect of the independent variable.
- Randomization: Ensures that participants are assigned to groups in a way that reduces bias, enhancing the validity of the results.
- Replication: Conducting the experiment multiple times to confirm the results and ensure reliability.
By understanding these components, researchers can design experiments that yield clear, actionable insights, paving the way for advancements in various fields.
Exploring Different Types of Experimental Design
Completely Randomized Design
In a completely randomized design, every participant or subject is assigned to a treatment group entirely by chance. This approach ensures that each subject has an equal opportunity to be placed in any group, minimizing bias. It's often used when the subjects are relatively homogeneous, meaning there aren't significant differences between them that could affect the outcome. This design is straightforward but can be limited if there are underlying variables that need to be controlled.
Randomized Block Design
The randomized block design is a bit more complex. Here, subjects are first divided into blocks or groups based on a specific characteristic, such as age or gender, which they share. Once the blocks are formed, participants within each block are randomly assigned to different treatments. This design helps control for variables that might affect the results, making it more precise than a completely randomized design. For instance, if you're studying the effect of a new drug, you might block by age to ensure that age differences don't skew your results.
Factorial Design
A factorial design is used when researchers want to study the effects of two or more variables at the same time. In this setup, every possible combination of the levels of the variables is tested. This design can provide a lot of information because it allows researchers to see not only the main effects of each variable but also how the variables interact with each other. However, it can become quite complex, especially as the number of variables increases. For example, if you're testing the impact of diet and exercise on health, a factorial design would let you see how each factor independently affects health, as well as how they work together.
By understanding these different designs, researchers can better tailor their studies to fit their specific needs and questions. It's all about choosing the right tool for the job, ensuring that the study is both effective and efficient.
Between-Subjects vs. Within-Subjects Designs
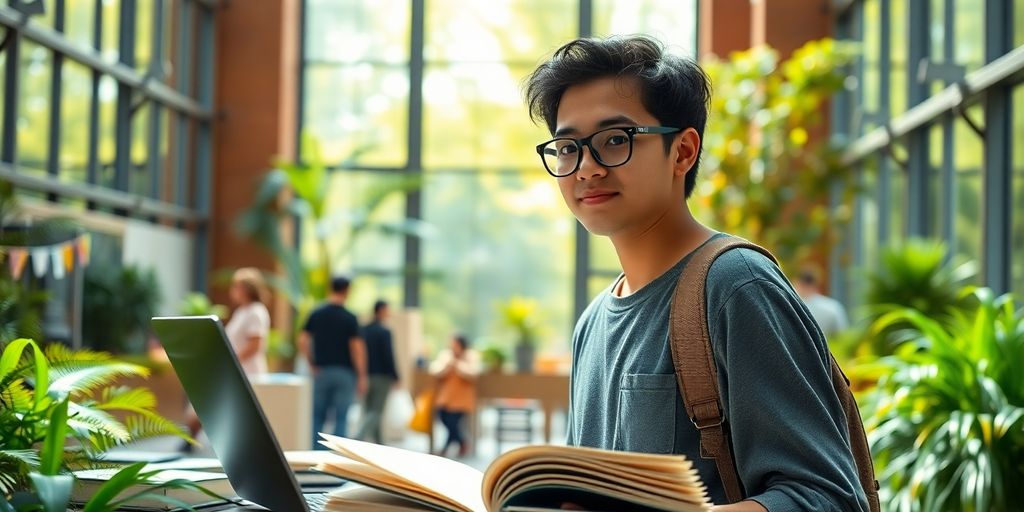
Characteristics of Between-Subjects Design
In a between-subjects design, participants are divided into different groups, and each group experiences a different condition of the experiment. This means that each participant is exposed to only one level of the independent variable. For example, if a study is testing the effectiveness of two different teaching methods, one group might experience Method A, while another group experiences Method B. This design is particularly useful when the treatment might have a lasting effect, making it difficult for participants to "unlearn" or "reset" between treatments.
Advantages and Disadvantages of Within-Subjects Design
A within-subjects design, on the other hand, involves the same participants experiencing all levels of the independent variable. This means that each participant acts as their own control, which can help reduce the variability due to individual differences. One major advantage of this design is that it generally requires fewer participants, as each one provides data for all conditions. However, it can also lead to issues such as order effects, where the sequence in which treatments are presented affects the results. To mitigate this, researchers often use counterbalancing to vary the order of treatments among participants.
Choosing Between the Two Designs
Deciding between these two designs often depends on the specific research question and practical considerations. Between-subjects designs are typically preferred when the treatment might have a permanent or long-lasting effect, or when there's a risk of participants becoming "test-wise" if they experience all conditions. In contrast, within-subjects designs are often favored when controlling for individual differences is crucial, or when resources are limited, as they require fewer participants. Ultimately, the choice hinges on balancing the need for rigorous control with the practicalities of conducting the study.
Single-Subject Experimental Designs
Reversal Design Explained
The reversal design, often referred to as the A-B-A or withdrawal design, is a straightforward approach used to establish experimental control. Initially, researchers collect baseline data, then introduce an intervention and observe the changes. Finally, the intervention is removed to see if the behavior returns to baseline levels. This design is excellent for demonstrating control because it clearly shows the effects of the intervention. However, it does have its drawbacks—ethical concerns arise when withdrawing an effective treatment, and some behaviors may not be reversible.
Multiple Baseline Design
When it's not feasible to withdraw a treatment, the multiple baseline design comes into play. This approach measures the effect of an intervention across different settings, behaviors, or individuals without removing the treatment. For instance, you might see its application in classrooms, home settings, and clinics simultaneously. Researchers introduce the intervention in a staggered manner across these contexts, ensuring that changes are due to the intervention and not external factors. This design is particularly useful when working with behaviors that cannot be reversed or when ethical considerations prevent withdrawal.
Alternating Treatments Design
The alternating treatments design allows researchers to compare two or more interventions quickly. By alternating between treatments in a random order, it becomes easier to determine which intervention is most effective. This design is beneficial when time is limited or when it's crucial to identify the best treatment among several options. However, frequent switching between treatments can sometimes lead to confusion, making it challenging to attribute changes to a specific intervention.
Advanced Experimental Design Techniques
Quasi-Experimental Design
Quasi-experimental designs are often used when true experimental conditions are difficult or impossible to achieve. They lack the random assignment of subjects to groups, which can introduce some biases. However, they are incredibly useful in real-world settings where randomization isn't feasible. For instance, when evaluating the impact of a new educational program in schools, it's often impractical to randomly assign students to different programs. Instead, researchers might compare outcomes between schools that have adopted the program and those that haven't. This approach helps in understanding the effects while acknowledging potential confounding variables.
Mixed-Methods Design
Mixed-methods design combines qualitative and quantitative research methods to provide a more comprehensive understanding of a research problem. This approach allows researchers to explore complex phenomena from multiple angles. For example, a study might use quantitative surveys to gather broad data about user satisfaction with Apple's innovative strategies and qualitative interviews to delve deeper into individual experiences. By integrating both methods, researchers can validate findings and gain richer insights. This design is particularly beneficial in fields like social sciences, where understanding human behavior requires both statistical data and personal narratives.
Longitudinal Design
Longitudinal designs involve studying the same subjects over an extended period, allowing researchers to observe changes and developments. This method is essential in studies where time-related changes are significant, such as tracking the progression of a disease or the impact of an intervention over years. For instance, a longitudinal study might examine how the use of WhatsApp influences communication patterns among teenagers over several years. One major advantage of this design is its ability to identify causal relationships by observing how variables change over time. However, these studies can be resource-intensive and require careful planning to maintain participant involvement.
Addressing Challenges in Experimental Design
Dealing with Confounding Variables
Confounding variables can be a real headache in experimental design. They are those pesky third variables that sneak in and mess up the relationship you're trying to study. Imagine you're testing a new Instagram feature to see if it boosts user engagement. If there's a major Instagram update happening at the same time, that could be a confounding variable. To tackle these, you need to identify them early and plan your study to minimize their impact. This might involve using control groups or randomization. By carefully planning, you can keep these confounders in check and ensure your results are more reliable.
Ensuring Internal and External Validity
Internal validity is all about making sure that the changes in your dependent variable are truly due to your independent variable and not some other factor. This is where controlling confounding variables comes into play again. On the other hand, external validity is about how well your findings can be generalized to other settings or groups. It's like testing a new study technique and wondering if it will work for everyone or just the group you studied. Balancing these two types of validity is tricky, but crucial. You might have to make trade-offs, but focusing on your study's goals can guide your decisions.
Ethical Considerations in Experimental Design
Ethics in experimental design isn't just about ticking boxes. It's about respecting participants and ensuring their well-being. Whether you're working on a medical trial or a study on how to write your thesis in 30 days, ethical guidelines must be followed. This means getting informed consent, ensuring confidentiality, and minimizing harm. Ethics can sometimes limit what you can do in your study, but they are non-negotiable. After all, the integrity of your research depends on it.
Practical Applications of Experimental Design
Experimental Design in Psychology
In psychology, experimental design is a cornerstone for understanding human behavior. Researchers often employ between-subjects designs to compare different groups, like testing new therapies against traditional methods. For instance, a study might explore the effects of a new therapy on anxiety levels by comparing a group receiving the therapy to a control group. This setup helps to isolate the treatment's impact on the dependent variable, such as anxiety reduction. Psychological experiments rely heavily on robust design to ensure findings are reliable and applicable.
Applications in Medical Research
Medical research frequently uses experimental designs to test new drugs or treatments. A common approach is the randomized controlled trial (RCT), where participants are randomly assigned to either the treatment or control group. This method helps minimize bias, providing clearer insights into the treatment's effectiveness. For example, a new medication might be tested for its ability to lower blood pressure, with results compared between those who received the drug and those who did not. Such designs are crucial for ensuring internal and external validity, making the findings trustworthy and applicable to broader populations.
Use in Social Sciences
In the social sciences, experimental design helps explore societal issues and human interactions. Researchers might use factorial designs to study multiple variables simultaneously, such as the impact of educational interventions on student performance across different demographics. This allows for a comprehensive analysis of how various factors interact and influence outcomes. By carefully structuring these experiments, social scientists can draw meaningful conclusions that inform policy and practice, contributing to a deeper understanding of complex social dynamics.
Experimental design is not just a concept; it has real-world uses that can make a difference in various fields. Whether you're in science, business, or education, understanding how to set up experiments can help you make better decisions and solve problems effectively. If you're curious about how to apply these ideas in your own work, visit our website for more tips and resources!
Conclusion
Choosing the right experimental design is like picking the right tool for a job. Each type has its own strengths and fits different kinds of research questions. Whether you're comparing treatments, analyzing components, or exploring functions, the design you choose will shape your study's outcomes. It's not just about following a set of rules; it's about understanding what your study needs and how best to meet those needs. By carefully considering your options and aligning them with your research goals, you can ensure that your study is both effective and meaningful. So, take the time to plan your design thoughtfully, and you'll be well on your way to conducting successful research.
Frequently Asked Questions
What is experimental design?
Experimental design is a plan for how to conduct a scientific study. It helps researchers test hypotheses by organizing how to collect and analyze data.
Why is experimental design important in research?
Experimental design is crucial because it ensures the results of a study are valid and reliable. It helps eliminate biases and confounding variables, leading to more accurate conclusions.
What is the difference between a completely randomized design and a randomized block design?
In a completely randomized design, subjects are randomly assigned to different groups. In a randomized block design, subjects are first grouped based on certain characteristics, and then randomly assigned within those groups.
How do between-subjects and within-subjects designs differ?
In a between-subjects design, different groups of people are tested under each condition. In a within-subjects design, the same group of people is tested under all conditions.
What are some challenges in experimental design?
Some challenges include dealing with confounding variables, ensuring the study has internal and external validity, and addressing ethical concerns.
Where can experimental design be applied?
Experimental design can be used in various fields like psychology, medicine, and social sciences to test hypotheses and draw conclusions from experiments.
Experimental Design Breakdown: Which Type Fits Your Study?

Ever found yourself tangled in the web of experimental design? You're not alone. It's like trying to choose the right tool from a massive toolbox without knowing what each does. Experimental design, at its core, is about structuring your research so you can get clear, reliable results. But with so many types out there, it can feel overwhelming. This article is here to break down the different types of experimental design and help you figure out which one suits your study best.
Key Takeaways
- Experimental design is essential for reliable research outcomes.
- Different designs suit different study needs and questions.
- Completely randomized designs are straightforward but may lack control over variables.
- Randomized block designs help account for variability within groups.
- Factorial designs allow for the study of multiple factors simultaneously.
Understanding the Foundations of Experimental Design
Defining Experimental Design
Experimental design is the blueprint for conducting research that seeks to establish cause-and-effect relationships. It involves the careful planning of how to manipulate one or more independent variables to observe their effects on dependent variables. This structured approach ensures that the results are reliable and can be attributed to the variables being tested, rather than external factors.
Importance of Experimental Design in Research
The significance of experimental design in research cannot be overstated. A well-structured experimental design enhances the credibility of a study by minimizing biases and errors. It provides a framework for testing hypotheses in a controlled environment, thereby yielding results that are both replicable and generalizable. For researchers, especially those sharing their findings on platforms like LinkedIn, a robust experimental design is essential for gaining trust and recognition in the academic community.
Key Components of Experimental Design
An effective experimental design comprises several key components:
- Variables: These include independent variables (manipulated by the researcher) and dependent variables (measured outcomes).
- Control Groups: Used to compare against experimental groups to ascertain the effect of the independent variable.
- Randomization: Ensures that participants are assigned to groups in a way that reduces bias, enhancing the validity of the results.
- Replication: Conducting the experiment multiple times to confirm the results and ensure reliability.
By understanding these components, researchers can design experiments that yield clear, actionable insights, paving the way for advancements in various fields.
Exploring Different Types of Experimental Design
Completely Randomized Design
In a completely randomized design, every participant or subject is assigned to a treatment group entirely by chance. This approach ensures that each subject has an equal opportunity to be placed in any group, minimizing bias. It's often used when the subjects are relatively homogeneous, meaning there aren't significant differences between them that could affect the outcome. This design is straightforward but can be limited if there are underlying variables that need to be controlled.
Randomized Block Design
The randomized block design is a bit more complex. Here, subjects are first divided into blocks or groups based on a specific characteristic, such as age or gender, which they share. Once the blocks are formed, participants within each block are randomly assigned to different treatments. This design helps control for variables that might affect the results, making it more precise than a completely randomized design. For instance, if you're studying the effect of a new drug, you might block by age to ensure that age differences don't skew your results.
Factorial Design
A factorial design is used when researchers want to study the effects of two or more variables at the same time. In this setup, every possible combination of the levels of the variables is tested. This design can provide a lot of information because it allows researchers to see not only the main effects of each variable but also how the variables interact with each other. However, it can become quite complex, especially as the number of variables increases. For example, if you're testing the impact of diet and exercise on health, a factorial design would let you see how each factor independently affects health, as well as how they work together.
By understanding these different designs, researchers can better tailor their studies to fit their specific needs and questions. It's all about choosing the right tool for the job, ensuring that the study is both effective and efficient.
Between-Subjects vs. Within-Subjects Designs
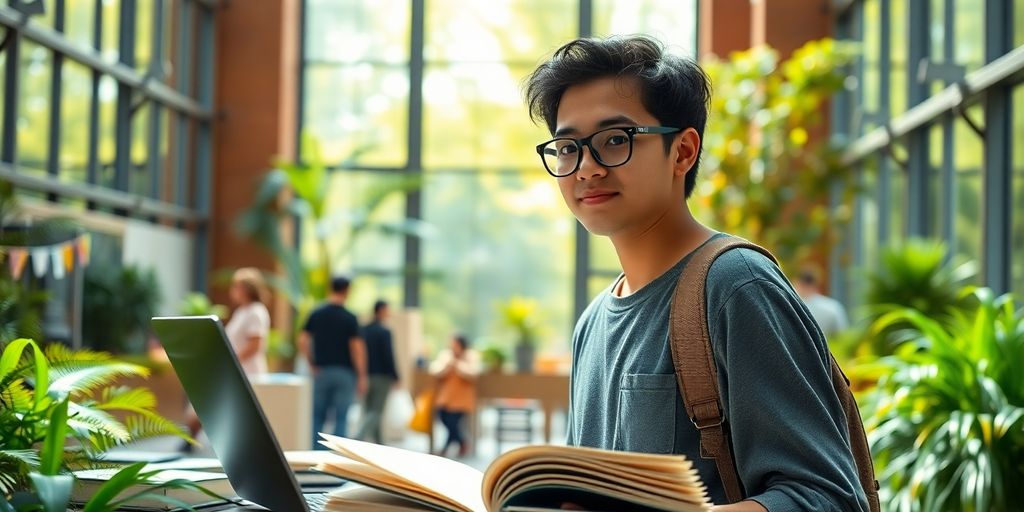
Characteristics of Between-Subjects Design
In a between-subjects design, participants are divided into different groups, and each group experiences a different condition of the experiment. This means that each participant is exposed to only one level of the independent variable. For example, if a study is testing the effectiveness of two different teaching methods, one group might experience Method A, while another group experiences Method B. This design is particularly useful when the treatment might have a lasting effect, making it difficult for participants to "unlearn" or "reset" between treatments.
Advantages and Disadvantages of Within-Subjects Design
A within-subjects design, on the other hand, involves the same participants experiencing all levels of the independent variable. This means that each participant acts as their own control, which can help reduce the variability due to individual differences. One major advantage of this design is that it generally requires fewer participants, as each one provides data for all conditions. However, it can also lead to issues such as order effects, where the sequence in which treatments are presented affects the results. To mitigate this, researchers often use counterbalancing to vary the order of treatments among participants.
Choosing Between the Two Designs
Deciding between these two designs often depends on the specific research question and practical considerations. Between-subjects designs are typically preferred when the treatment might have a permanent or long-lasting effect, or when there's a risk of participants becoming "test-wise" if they experience all conditions. In contrast, within-subjects designs are often favored when controlling for individual differences is crucial, or when resources are limited, as they require fewer participants. Ultimately, the choice hinges on balancing the need for rigorous control with the practicalities of conducting the study.
Single-Subject Experimental Designs
Reversal Design Explained
The reversal design, often referred to as the A-B-A or withdrawal design, is a straightforward approach used to establish experimental control. Initially, researchers collect baseline data, then introduce an intervention and observe the changes. Finally, the intervention is removed to see if the behavior returns to baseline levels. This design is excellent for demonstrating control because it clearly shows the effects of the intervention. However, it does have its drawbacks—ethical concerns arise when withdrawing an effective treatment, and some behaviors may not be reversible.
Multiple Baseline Design
When it's not feasible to withdraw a treatment, the multiple baseline design comes into play. This approach measures the effect of an intervention across different settings, behaviors, or individuals without removing the treatment. For instance, you might see its application in classrooms, home settings, and clinics simultaneously. Researchers introduce the intervention in a staggered manner across these contexts, ensuring that changes are due to the intervention and not external factors. This design is particularly useful when working with behaviors that cannot be reversed or when ethical considerations prevent withdrawal.
Alternating Treatments Design
The alternating treatments design allows researchers to compare two or more interventions quickly. By alternating between treatments in a random order, it becomes easier to determine which intervention is most effective. This design is beneficial when time is limited or when it's crucial to identify the best treatment among several options. However, frequent switching between treatments can sometimes lead to confusion, making it challenging to attribute changes to a specific intervention.
Advanced Experimental Design Techniques
Quasi-Experimental Design
Quasi-experimental designs are often used when true experimental conditions are difficult or impossible to achieve. They lack the random assignment of subjects to groups, which can introduce some biases. However, they are incredibly useful in real-world settings where randomization isn't feasible. For instance, when evaluating the impact of a new educational program in schools, it's often impractical to randomly assign students to different programs. Instead, researchers might compare outcomes between schools that have adopted the program and those that haven't. This approach helps in understanding the effects while acknowledging potential confounding variables.
Mixed-Methods Design
Mixed-methods design combines qualitative and quantitative research methods to provide a more comprehensive understanding of a research problem. This approach allows researchers to explore complex phenomena from multiple angles. For example, a study might use quantitative surveys to gather broad data about user satisfaction with Apple's innovative strategies and qualitative interviews to delve deeper into individual experiences. By integrating both methods, researchers can validate findings and gain richer insights. This design is particularly beneficial in fields like social sciences, where understanding human behavior requires both statistical data and personal narratives.
Longitudinal Design
Longitudinal designs involve studying the same subjects over an extended period, allowing researchers to observe changes and developments. This method is essential in studies where time-related changes are significant, such as tracking the progression of a disease or the impact of an intervention over years. For instance, a longitudinal study might examine how the use of WhatsApp influences communication patterns among teenagers over several years. One major advantage of this design is its ability to identify causal relationships by observing how variables change over time. However, these studies can be resource-intensive and require careful planning to maintain participant involvement.
Addressing Challenges in Experimental Design
Dealing with Confounding Variables
Confounding variables can be a real headache in experimental design. They are those pesky third variables that sneak in and mess up the relationship you're trying to study. Imagine you're testing a new Instagram feature to see if it boosts user engagement. If there's a major Instagram update happening at the same time, that could be a confounding variable. To tackle these, you need to identify them early and plan your study to minimize their impact. This might involve using control groups or randomization. By carefully planning, you can keep these confounders in check and ensure your results are more reliable.
Ensuring Internal and External Validity
Internal validity is all about making sure that the changes in your dependent variable are truly due to your independent variable and not some other factor. This is where controlling confounding variables comes into play again. On the other hand, external validity is about how well your findings can be generalized to other settings or groups. It's like testing a new study technique and wondering if it will work for everyone or just the group you studied. Balancing these two types of validity is tricky, but crucial. You might have to make trade-offs, but focusing on your study's goals can guide your decisions.
Ethical Considerations in Experimental Design
Ethics in experimental design isn't just about ticking boxes. It's about respecting participants and ensuring their well-being. Whether you're working on a medical trial or a study on how to write your thesis in 30 days, ethical guidelines must be followed. This means getting informed consent, ensuring confidentiality, and minimizing harm. Ethics can sometimes limit what you can do in your study, but they are non-negotiable. After all, the integrity of your research depends on it.
Practical Applications of Experimental Design
Experimental Design in Psychology
In psychology, experimental design is a cornerstone for understanding human behavior. Researchers often employ between-subjects designs to compare different groups, like testing new therapies against traditional methods. For instance, a study might explore the effects of a new therapy on anxiety levels by comparing a group receiving the therapy to a control group. This setup helps to isolate the treatment's impact on the dependent variable, such as anxiety reduction. Psychological experiments rely heavily on robust design to ensure findings are reliable and applicable.
Applications in Medical Research
Medical research frequently uses experimental designs to test new drugs or treatments. A common approach is the randomized controlled trial (RCT), where participants are randomly assigned to either the treatment or control group. This method helps minimize bias, providing clearer insights into the treatment's effectiveness. For example, a new medication might be tested for its ability to lower blood pressure, with results compared between those who received the drug and those who did not. Such designs are crucial for ensuring internal and external validity, making the findings trustworthy and applicable to broader populations.
Use in Social Sciences
In the social sciences, experimental design helps explore societal issues and human interactions. Researchers might use factorial designs to study multiple variables simultaneously, such as the impact of educational interventions on student performance across different demographics. This allows for a comprehensive analysis of how various factors interact and influence outcomes. By carefully structuring these experiments, social scientists can draw meaningful conclusions that inform policy and practice, contributing to a deeper understanding of complex social dynamics.
Experimental design is not just a concept; it has real-world uses that can make a difference in various fields. Whether you're in science, business, or education, understanding how to set up experiments can help you make better decisions and solve problems effectively. If you're curious about how to apply these ideas in your own work, visit our website for more tips and resources!
Conclusion
Choosing the right experimental design is like picking the right tool for a job. Each type has its own strengths and fits different kinds of research questions. Whether you're comparing treatments, analyzing components, or exploring functions, the design you choose will shape your study's outcomes. It's not just about following a set of rules; it's about understanding what your study needs and how best to meet those needs. By carefully considering your options and aligning them with your research goals, you can ensure that your study is both effective and meaningful. So, take the time to plan your design thoughtfully, and you'll be well on your way to conducting successful research.
Frequently Asked Questions
What is experimental design?
Experimental design is a plan for how to conduct a scientific study. It helps researchers test hypotheses by organizing how to collect and analyze data.
Why is experimental design important in research?
Experimental design is crucial because it ensures the results of a study are valid and reliable. It helps eliminate biases and confounding variables, leading to more accurate conclusions.
What is the difference between a completely randomized design and a randomized block design?
In a completely randomized design, subjects are randomly assigned to different groups. In a randomized block design, subjects are first grouped based on certain characteristics, and then randomly assigned within those groups.
How do between-subjects and within-subjects designs differ?
In a between-subjects design, different groups of people are tested under each condition. In a within-subjects design, the same group of people is tested under all conditions.
What are some challenges in experimental design?
Some challenges include dealing with confounding variables, ensuring the study has internal and external validity, and addressing ethical concerns.
Where can experimental design be applied?
Experimental design can be used in various fields like psychology, medicine, and social sciences to test hypotheses and draw conclusions from experiments.
Experimental Design Breakdown: Which Type Fits Your Study?

Ever found yourself tangled in the web of experimental design? You're not alone. It's like trying to choose the right tool from a massive toolbox without knowing what each does. Experimental design, at its core, is about structuring your research so you can get clear, reliable results. But with so many types out there, it can feel overwhelming. This article is here to break down the different types of experimental design and help you figure out which one suits your study best.
Key Takeaways
- Experimental design is essential for reliable research outcomes.
- Different designs suit different study needs and questions.
- Completely randomized designs are straightforward but may lack control over variables.
- Randomized block designs help account for variability within groups.
- Factorial designs allow for the study of multiple factors simultaneously.
Understanding the Foundations of Experimental Design
Defining Experimental Design
Experimental design is the blueprint for conducting research that seeks to establish cause-and-effect relationships. It involves the careful planning of how to manipulate one or more independent variables to observe their effects on dependent variables. This structured approach ensures that the results are reliable and can be attributed to the variables being tested, rather than external factors.
Importance of Experimental Design in Research
The significance of experimental design in research cannot be overstated. A well-structured experimental design enhances the credibility of a study by minimizing biases and errors. It provides a framework for testing hypotheses in a controlled environment, thereby yielding results that are both replicable and generalizable. For researchers, especially those sharing their findings on platforms like LinkedIn, a robust experimental design is essential for gaining trust and recognition in the academic community.
Key Components of Experimental Design
An effective experimental design comprises several key components:
- Variables: These include independent variables (manipulated by the researcher) and dependent variables (measured outcomes).
- Control Groups: Used to compare against experimental groups to ascertain the effect of the independent variable.
- Randomization: Ensures that participants are assigned to groups in a way that reduces bias, enhancing the validity of the results.
- Replication: Conducting the experiment multiple times to confirm the results and ensure reliability.
By understanding these components, researchers can design experiments that yield clear, actionable insights, paving the way for advancements in various fields.
Exploring Different Types of Experimental Design
Completely Randomized Design
In a completely randomized design, every participant or subject is assigned to a treatment group entirely by chance. This approach ensures that each subject has an equal opportunity to be placed in any group, minimizing bias. It's often used when the subjects are relatively homogeneous, meaning there aren't significant differences between them that could affect the outcome. This design is straightforward but can be limited if there are underlying variables that need to be controlled.
Randomized Block Design
The randomized block design is a bit more complex. Here, subjects are first divided into blocks or groups based on a specific characteristic, such as age or gender, which they share. Once the blocks are formed, participants within each block are randomly assigned to different treatments. This design helps control for variables that might affect the results, making it more precise than a completely randomized design. For instance, if you're studying the effect of a new drug, you might block by age to ensure that age differences don't skew your results.
Factorial Design
A factorial design is used when researchers want to study the effects of two or more variables at the same time. In this setup, every possible combination of the levels of the variables is tested. This design can provide a lot of information because it allows researchers to see not only the main effects of each variable but also how the variables interact with each other. However, it can become quite complex, especially as the number of variables increases. For example, if you're testing the impact of diet and exercise on health, a factorial design would let you see how each factor independently affects health, as well as how they work together.
By understanding these different designs, researchers can better tailor their studies to fit their specific needs and questions. It's all about choosing the right tool for the job, ensuring that the study is both effective and efficient.
Between-Subjects vs. Within-Subjects Designs
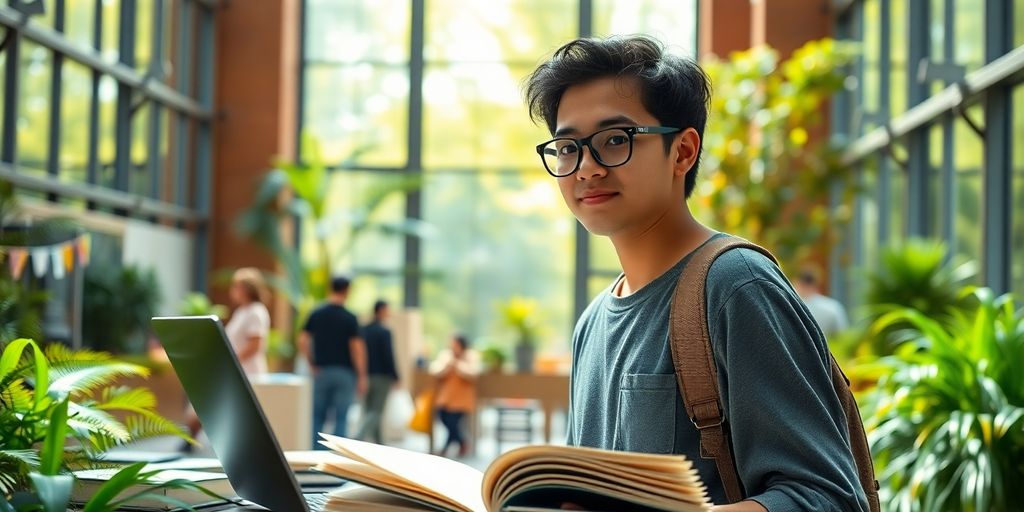
Characteristics of Between-Subjects Design
In a between-subjects design, participants are divided into different groups, and each group experiences a different condition of the experiment. This means that each participant is exposed to only one level of the independent variable. For example, if a study is testing the effectiveness of two different teaching methods, one group might experience Method A, while another group experiences Method B. This design is particularly useful when the treatment might have a lasting effect, making it difficult for participants to "unlearn" or "reset" between treatments.
Advantages and Disadvantages of Within-Subjects Design
A within-subjects design, on the other hand, involves the same participants experiencing all levels of the independent variable. This means that each participant acts as their own control, which can help reduce the variability due to individual differences. One major advantage of this design is that it generally requires fewer participants, as each one provides data for all conditions. However, it can also lead to issues such as order effects, where the sequence in which treatments are presented affects the results. To mitigate this, researchers often use counterbalancing to vary the order of treatments among participants.
Choosing Between the Two Designs
Deciding between these two designs often depends on the specific research question and practical considerations. Between-subjects designs are typically preferred when the treatment might have a permanent or long-lasting effect, or when there's a risk of participants becoming "test-wise" if they experience all conditions. In contrast, within-subjects designs are often favored when controlling for individual differences is crucial, or when resources are limited, as they require fewer participants. Ultimately, the choice hinges on balancing the need for rigorous control with the practicalities of conducting the study.
Single-Subject Experimental Designs
Reversal Design Explained
The reversal design, often referred to as the A-B-A or withdrawal design, is a straightforward approach used to establish experimental control. Initially, researchers collect baseline data, then introduce an intervention and observe the changes. Finally, the intervention is removed to see if the behavior returns to baseline levels. This design is excellent for demonstrating control because it clearly shows the effects of the intervention. However, it does have its drawbacks—ethical concerns arise when withdrawing an effective treatment, and some behaviors may not be reversible.
Multiple Baseline Design
When it's not feasible to withdraw a treatment, the multiple baseline design comes into play. This approach measures the effect of an intervention across different settings, behaviors, or individuals without removing the treatment. For instance, you might see its application in classrooms, home settings, and clinics simultaneously. Researchers introduce the intervention in a staggered manner across these contexts, ensuring that changes are due to the intervention and not external factors. This design is particularly useful when working with behaviors that cannot be reversed or when ethical considerations prevent withdrawal.
Alternating Treatments Design
The alternating treatments design allows researchers to compare two or more interventions quickly. By alternating between treatments in a random order, it becomes easier to determine which intervention is most effective. This design is beneficial when time is limited or when it's crucial to identify the best treatment among several options. However, frequent switching between treatments can sometimes lead to confusion, making it challenging to attribute changes to a specific intervention.
Advanced Experimental Design Techniques
Quasi-Experimental Design
Quasi-experimental designs are often used when true experimental conditions are difficult or impossible to achieve. They lack the random assignment of subjects to groups, which can introduce some biases. However, they are incredibly useful in real-world settings where randomization isn't feasible. For instance, when evaluating the impact of a new educational program in schools, it's often impractical to randomly assign students to different programs. Instead, researchers might compare outcomes between schools that have adopted the program and those that haven't. This approach helps in understanding the effects while acknowledging potential confounding variables.
Mixed-Methods Design
Mixed-methods design combines qualitative and quantitative research methods to provide a more comprehensive understanding of a research problem. This approach allows researchers to explore complex phenomena from multiple angles. For example, a study might use quantitative surveys to gather broad data about user satisfaction with Apple's innovative strategies and qualitative interviews to delve deeper into individual experiences. By integrating both methods, researchers can validate findings and gain richer insights. This design is particularly beneficial in fields like social sciences, where understanding human behavior requires both statistical data and personal narratives.
Longitudinal Design
Longitudinal designs involve studying the same subjects over an extended period, allowing researchers to observe changes and developments. This method is essential in studies where time-related changes are significant, such as tracking the progression of a disease or the impact of an intervention over years. For instance, a longitudinal study might examine how the use of WhatsApp influences communication patterns among teenagers over several years. One major advantage of this design is its ability to identify causal relationships by observing how variables change over time. However, these studies can be resource-intensive and require careful planning to maintain participant involvement.
Addressing Challenges in Experimental Design
Dealing with Confounding Variables
Confounding variables can be a real headache in experimental design. They are those pesky third variables that sneak in and mess up the relationship you're trying to study. Imagine you're testing a new Instagram feature to see if it boosts user engagement. If there's a major Instagram update happening at the same time, that could be a confounding variable. To tackle these, you need to identify them early and plan your study to minimize their impact. This might involve using control groups or randomization. By carefully planning, you can keep these confounders in check and ensure your results are more reliable.
Ensuring Internal and External Validity
Internal validity is all about making sure that the changes in your dependent variable are truly due to your independent variable and not some other factor. This is where controlling confounding variables comes into play again. On the other hand, external validity is about how well your findings can be generalized to other settings or groups. It's like testing a new study technique and wondering if it will work for everyone or just the group you studied. Balancing these two types of validity is tricky, but crucial. You might have to make trade-offs, but focusing on your study's goals can guide your decisions.
Ethical Considerations in Experimental Design
Ethics in experimental design isn't just about ticking boxes. It's about respecting participants and ensuring their well-being. Whether you're working on a medical trial or a study on how to write your thesis in 30 days, ethical guidelines must be followed. This means getting informed consent, ensuring confidentiality, and minimizing harm. Ethics can sometimes limit what you can do in your study, but they are non-negotiable. After all, the integrity of your research depends on it.
Practical Applications of Experimental Design
Experimental Design in Psychology
In psychology, experimental design is a cornerstone for understanding human behavior. Researchers often employ between-subjects designs to compare different groups, like testing new therapies against traditional methods. For instance, a study might explore the effects of a new therapy on anxiety levels by comparing a group receiving the therapy to a control group. This setup helps to isolate the treatment's impact on the dependent variable, such as anxiety reduction. Psychological experiments rely heavily on robust design to ensure findings are reliable and applicable.
Applications in Medical Research
Medical research frequently uses experimental designs to test new drugs or treatments. A common approach is the randomized controlled trial (RCT), where participants are randomly assigned to either the treatment or control group. This method helps minimize bias, providing clearer insights into the treatment's effectiveness. For example, a new medication might be tested for its ability to lower blood pressure, with results compared between those who received the drug and those who did not. Such designs are crucial for ensuring internal and external validity, making the findings trustworthy and applicable to broader populations.
Use in Social Sciences
In the social sciences, experimental design helps explore societal issues and human interactions. Researchers might use factorial designs to study multiple variables simultaneously, such as the impact of educational interventions on student performance across different demographics. This allows for a comprehensive analysis of how various factors interact and influence outcomes. By carefully structuring these experiments, social scientists can draw meaningful conclusions that inform policy and practice, contributing to a deeper understanding of complex social dynamics.
Experimental design is not just a concept; it has real-world uses that can make a difference in various fields. Whether you're in science, business, or education, understanding how to set up experiments can help you make better decisions and solve problems effectively. If you're curious about how to apply these ideas in your own work, visit our website for more tips and resources!
Conclusion
Choosing the right experimental design is like picking the right tool for a job. Each type has its own strengths and fits different kinds of research questions. Whether you're comparing treatments, analyzing components, or exploring functions, the design you choose will shape your study's outcomes. It's not just about following a set of rules; it's about understanding what your study needs and how best to meet those needs. By carefully considering your options and aligning them with your research goals, you can ensure that your study is both effective and meaningful. So, take the time to plan your design thoughtfully, and you'll be well on your way to conducting successful research.
Frequently Asked Questions
What is experimental design?
Experimental design is a plan for how to conduct a scientific study. It helps researchers test hypotheses by organizing how to collect and analyze data.
Why is experimental design important in research?
Experimental design is crucial because it ensures the results of a study are valid and reliable. It helps eliminate biases and confounding variables, leading to more accurate conclusions.
What is the difference between a completely randomized design and a randomized block design?
In a completely randomized design, subjects are randomly assigned to different groups. In a randomized block design, subjects are first grouped based on certain characteristics, and then randomly assigned within those groups.
How do between-subjects and within-subjects designs differ?
In a between-subjects design, different groups of people are tested under each condition. In a within-subjects design, the same group of people is tested under all conditions.
What are some challenges in experimental design?
Some challenges include dealing with confounding variables, ensuring the study has internal and external validity, and addressing ethical concerns.
Where can experimental design be applied?
Experimental design can be used in various fields like psychology, medicine, and social sciences to test hypotheses and draw conclusions from experiments.