Master Quasi-Experimental Research Design for Real-World Applications

So, you're diving into the world of quasi-experimental research design, huh? It's one of those things that sounds super complicated, but once you get the hang of it, it can be a game-changer. This type of research is like a middle ground between full-blown experiments and just observing stuff. You don't get to randomly assign people or things to groups, which can be a bit of a bummer, but it's perfect for real-world situations where you can't just shuffle things around like a deck of cards. You still get to play around with variables and see what happens, which is pretty cool.
Key Takeaways
- Quasi-experimental designs are great for real-world research where random assignments aren't possible.
- These designs help in studying cause-and-effect relationships without the strict controls of true experiments.
- They are widely used in fields like social sciences, policy evaluation, and market research.
- Quasi-experimental research can be more practical and ethical compared to true experiments.
- While they offer flexibility, these designs require careful consideration of confounding variables.
Understanding Quasi-Experimental Research Design
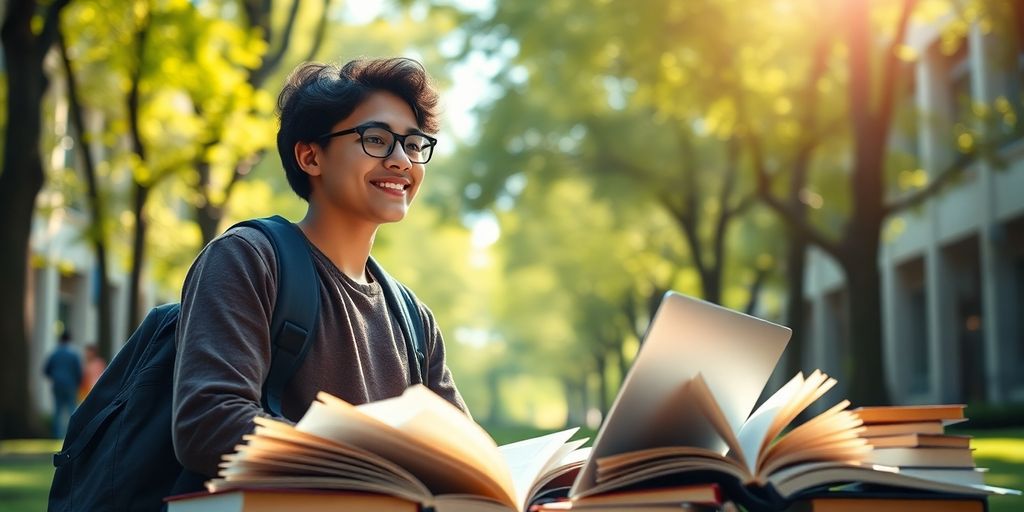
Defining Quasi-Experimental Design
A quasi-experimental design is a research method that shares similarities with true experimental design but differs in one critical aspect: the lack of random assignment. This approach is often chosen when it's impractical or unethical to randomly assign participants to different groups. In such studies, researchers manipulate an independent variable to observe its effect on a dependent variable, despite not having full control over the experimental conditions. This lack of randomization can lead to challenges in confidently attributing causality, yet it allows for real-world applications that might not be feasible otherwise.
Key Characteristics of Quasi-Experimental Research
Quasi-experimental research is marked by several defining traits:
- Absence of Randomization: Participants are not randomly assigned to treatment or control groups, often relying on naturally occurring groups instead.
- Manipulation of Variables: Despite the absence of randomization, researchers still intervene by introducing a treatment condition, aiming to measure its impact.
- Comparison Groups: Typically involves a treatment group and a control group, though these are not formed through random assignment.
- Mitigation of Confounding Variables: Researchers need to address potential confounding variables that might influence the outcome.
Distinguishing Features from True Experimental Design
While both true experimental and quasi-experimental designs involve manipulation of variables, the key difference lies in the assignment of participants. True experiments use random assignment, providing greater internal validity and stronger causal inferences. In contrast, quasi-experiments lack this randomization, which can introduce bias but offers greater external validity due to their application in more natural settings. This makes them particularly useful in fields like social science and policy evaluation, where choosing the right study design is crucial for effective research outcomes. Despite their limitations, quasi-experimental designs remain a valuable tool for researchers seeking to explore causal relationships in complex, real-world environments, much like how to write your thesis in 30 days requires a structured and adaptable approach.
Applications of Quasi-Experimental Design in Real-World Research
Quasi-experimental designs are a staple in social science research, allowing researchers to explore causal relationships in settings where traditional experiments aren't feasible. These designs are invaluable when ethical or practical constraints prevent random assignment. For example, when studying the impact of educational interventions, researchers might use existing class assignments as groups, comparing outcomes between those exposed to a new teaching method and those who are not. This approach can reveal significant insights into educational practices and policy impacts.
Implementing Quasi-Experimental Methods in Policy Evaluation
In policy evaluation, quasi-experimental designs help assess the effectiveness of government programs and initiatives. They enable the examination of outcomes before and after policy implementation, using methods like interrupted time-series analysis to identify changes attributable to the policy. This can be particularly useful in evaluating public health interventions, where randomization may not be possible. By comparing regions with and without the policy, researchers can infer the policy's impact, providing data-driven insights for future decision-making.
Market Research and Quasi-Experimental Approaches
Market researchers often turn to quasi-experimental designs to evaluate the success of marketing strategies and consumer behavior. For instance, when introducing a new product, businesses might compare sales trends in areas where the product is launched against those where it's not. Similarly, non-experimental research methods can be used to assess the impact of advertising campaigns by analyzing changes in brand awareness or sales. This method allows firms to gauge the effectiveness of their strategies without the need for costly and time-consuming randomized trials. By leveraging existing data and naturally occurring groups, companies can make informed decisions that directly influence their marketing tactics.
Types of Quasi-Experimental Designs
When diving into the realm of quasi-experimental research, it's essential to understand the different types of designs available. Each type offers unique ways to tackle research questions, especially when random assignment is not feasible. Let's explore some of the main quasi-experimental designs.
Nonequivalent Groups Design
The nonequivalent groups design is one of the most common forms used in quasi-experimental research. In this setup, researchers compare outcomes between groups that have not been randomly assigned. Imagine you want to study the effectiveness of a new teaching method. You might have one class use the new method while another class continues with the traditional approach. Both groups are given a pretest and a posttest to measure the impact. The key here is that the groups are as similar as possible, but without random assignment, they might still differ in ways that could affect the results.
Regression Discontinuity Design
Regression discontinuity design is a bit more complex but offers a clever way to assess causal effects. It involves assigning participants to different groups based on a cutoff score on a pre-treatment variable. For example, students might receive additional tutoring if their test scores fall below a certain threshold. By comparing those just above and below the cutoff, researchers can estimate the treatment's effect. This design helps in situations where ethical or practical reasons prevent random assignment.
Interrupted Time Series Design
The interrupted time series design is all about tracking changes over time. Researchers collect data at multiple intervals before and after an intervention. Let's say a new law is implemented to reduce traffic accidents. By analyzing accident rates before and after the law's enactment, researchers can infer its impact. This design is powerful for assessing long-term effects, though it requires a substantial amount of data and careful consideration of external factors that might influence the results.
In conclusion, understanding these designs is crucial for anyone conducting quasi-experimental studies. They provide flexibility in real-world settings where traditional experimental methods might not be applicable. Each design has its strengths and limitations, making it vital to choose the one that best fits your research context.
Advantages and Limitations of Quasi-Experimental Research
Benefits of Quasi-Experimental Design
Quasi-experimental research is like a Swiss Army knife for researchers. It's super handy when you can't use a true experimental design. One of its biggest perks is how well it fits into real-world scenarios, especially when dealing with social issues, policies, or market trends. Imagine trying to test the impact of WhatsApp on communication habits without being able to randomly assign people to use it or not. That's where quasi-experiments shine.
Here are a few reasons why researchers love this approach:
- Real-world applicability: You can study events or phenomena as they naturally occur, like policy changes or natural disasters, without needing to manipulate the environment artificially.
- Higher external validity: Since these studies often happen in natural settings, the findings tend to be more relatable to the real world.
- Cost-effective: Quasi-experiments often use existing data or naturally occurring groups, saving time and money compared to setting up a full-blown experiment.
Challenges and Limitations
But it's not all sunshine and rainbows. Quasi-experimental designs come with their own set of challenges. The biggest headache? Lower internal validity. Without random assignment, it's tough to rule out other reasons for your results. Maybe the people using WhatsApp were already better at communicating.
Here's what researchers have to watch out for:
- Selection bias: Pre-existing differences between groups can mess with the results. It's like comparing apples to oranges.
- Confounding variables: These pesky variables can sneak in and affect the outcome, making it hard to pinpoint what's really going on.
- Regression to the mean: Especially in pretest-posttest setups, this can lead to wrong conclusions about what's causing changes.
Balancing Internal and External Validity
Finding the sweet spot between internal and external validity is a balancing act. Internal validity is all about making sure your results are due to the treatment, while external validity is about how well those results apply outside the study.
In quasi-experimental research, you often have to trade a bit of internal validity for the sake of external validity. It's like trying to balance on a seesaw; you can't have both sides perfectly level. But with careful planning and statistical techniques, researchers can still draw meaningful conclusions.
In the end, quasi-experimental designs are a practical choice when randomization isn't possible. They allow for meaningful insights into real-world phenomena, like how WhatsApp influences communication patterns, despite their limitations.
Methodological Considerations in Quasi-Experimental Research
Addressing Confounding Variables
When diving into quasi-experimental research, one of the first hurdles is dealing with confounding variables. These are the pesky factors that can skew results if not handled properly. Unlike true experiments, where random assignment can help balance these out, quasi-experiments have to get creative. Researchers often use techniques like matching or statistical controls to level the playing field. Imagine you're studying the impact of a new teaching method in schools. If one school has newer facilities, that could influence the results. Adjusting for such variables is key to making sure the findings are valid.
Ensuring Validity and Reliability
Ensuring that your research is both valid and reliable is like making sure your Instagram feed is both trendy and consistent. Validity means you're actually measuring what you think you're measuring. Reliability is about getting the same results if you did the study again. In quasi-experimental designs, this can be tricky. Researchers often rely on repeated measures or triangulation, using multiple methods or data sources to confirm findings. This way, they can be more confident that their results are not just a fluke.
Ethical Considerations in Design
Ethical concerns are always a hot topic, and in quasi-experimental research, they can be quite the balancing act. Since random assignment isn't used, researchers must be extra careful about how participants are selected and treated. It's crucial to ensure that no group is unfairly advantaged or disadvantaged by the study. For instance, if a study involves a new health intervention, researchers must ensure that those not receiving the intervention aren't at risk. Transparency with participants about the nature of the study and its potential impacts is also a must.
Case Studies and Examples of Quasi-Experimental Design
In education, quasi-experimental designs are often used to evaluate the impact of new teaching methods or educational programs. For instance, a school district might introduce a new curriculum in select schools while maintaining the existing one in others. This setup allows researchers to compare student performance across different teaching methods without random assignment. Such studies can reveal insights into educational practices that might be more effective for certain student populations.
Public Health Applications
Quasi-experimental designs are also prevalent in public health research. They help assess the effects of health interventions when randomized trials aren't feasible due to ethical or logistical reasons. For example, a city might implement a smoking cessation program in certain neighborhoods and use others as a control. Researchers can then compare health outcomes like smoking rates or respiratory health between these areas, offering valuable data on the program's effectiveness.
Market Research Case Studies
In the realm of market research, quasi-experimental designs offer a practical way to test hypotheses about consumer behavior. Consider a retail chain assessing a new store layout's impact on sales. Stores with the new layout serve as the experimental group, while those with the old design act as the control. Over time, sales data from both groups are analyzed to determine the layout's effectiveness. Another example includes an online retailer using a regression discontinuity design to evaluate a loyalty program by comparing purchasing behavior just above and below a spending threshold. These approaches allow businesses to make informed decisions based on real-world data.
For those interested in exploring past events to understand their impacts, ex post facto research provides a method to analyze relationships between variables through observational data, offering insights into policy development and behavioral influences.
Statistical Techniques in Quasi-Experimental Research
Quasi-experimental research is a fascinating field, offering a middle ground between true experiments and observational studies. While it lacks the rigor of random assignment, it compensates with real-world applicability. To make the most of this design, researchers often rely on a variety of statistical techniques. Here, we'll explore some of the most common methods used to analyze quasi-experimental data.
Propensity Score Matching
Propensity score matching is a statistical method used to create a balanced comparison between groups in a study. This technique attempts to mimic random assignment by matching participants based on similar characteristics. By doing so, it helps control for confounding variables and reduces bias, making it easier to identify the treatment effect. This method is particularly useful in studies where randomization isn't feasible.
Regression Analysis in Quasi-Experiments
Regression analysis is another powerful tool in the arsenal of quasi-experimental research. It allows researchers to assess the relationship between variables and control for potential confounders. In this context, multiple regression can be used to adjust for various factors that might influence the outcome. By incorporating these variables into the model, researchers can better isolate the effect of the treatment.
Handling Missing Data
Missing data is a common issue in any research, and quasi-experimental studies are no exception. Techniques such as multiple imputation and maximum likelihood estimation are often employed to address this problem. These methods help to fill in the gaps, ensuring that the analysis remains robust and reliable. Handling missing data properly is crucial to maintaining the integrity of the study's findings.
In summary, while quasi-experimental designs may not offer the same level of control as true experiments, they provide valuable insights into causal relationships in real-world settings. By employing statistical techniques like propensity score matching, regression analysis, and methods for handling missing data, researchers can enhance the validity and reliability of their findings.
In quasi-experimental research, statistical methods play a key role in understanding data. These techniques help researchers make sense of their findings and draw meaningful conclusions. If you're a student feeling overwhelmed by your thesis, don't worry! Visit our website to discover how our Thesis Action Plan can guide you through every step of the writing process. Take control of your academic journey today!
Conclusion
In wrapping up our exploration of quasi-experimental research design, it's clear that this approach offers a practical solution for real-world research challenges. While it doesn't boast the same level of control as true experimental designs, its flexibility and applicability in natural settings make it invaluable. By allowing researchers to study causal relationships without the constraints of randomization, quasi-experimental designs open doors to insights that might otherwise remain unexplored. Whether in social sciences, policy evaluation, or market research, this method provides a robust framework for understanding complex phenomena. As we continue to refine these techniques, the potential for meaningful discoveries only grows. Embracing the strengths and acknowledging the limitations of quasi-experimental designs will ensure they remain a vital tool in the researcher's toolkit.
Frequently Asked Questions
What exactly is a quasi-experimental design?
A quasi-experimental design is a research method that looks a lot like a real experiment but doesn't use random assignment. Researchers study how one thing affects another in real-life settings without randomly choosing who gets what treatment.
How does a quasi-experimental design differ from a true experiment?
The big difference is in how participants are assigned to groups. In true experiments, participants are randomly assigned to groups, but in quasi-experiments, they aren't. This means quasi-experiments might have more biases, but they can still be useful.
Why would someone choose a quasi-experimental design over a true experiment?
Sometimes it's not possible or ethical to randomly assign people to groups, like when studying the effects of a new teaching method in schools. Quasi-experimental designs let researchers study real-world situations without needing random assignment.
Can you give an example of a quasi-experimental design?
Sure! Imagine a study that looks at how a new reading program affects students' test scores. Instead of randomly assigning students to use the new program, researchers might compare scores from two existing classes, one using the program and one not.
What are the advantages of using a quasi-experimental design?
Quasi-experimental designs are great for studying real-world situations where random assignment isn't possible. They offer more flexibility and can be less costly and time-consuming than true experiments.
Are there any downsides to quasi-experimental designs?
Yes, because they don't use random assignment, they might have more biases and less control over variables. This can make it harder to be sure that the treatment caused the effects seen in the study.
Master Quasi-Experimental Research Design for Real-World Applications

So, you're diving into the world of quasi-experimental research design, huh? It's one of those things that sounds super complicated, but once you get the hang of it, it can be a game-changer. This type of research is like a middle ground between full-blown experiments and just observing stuff. You don't get to randomly assign people or things to groups, which can be a bit of a bummer, but it's perfect for real-world situations where you can't just shuffle things around like a deck of cards. You still get to play around with variables and see what happens, which is pretty cool.
Key Takeaways
- Quasi-experimental designs are great for real-world research where random assignments aren't possible.
- These designs help in studying cause-and-effect relationships without the strict controls of true experiments.
- They are widely used in fields like social sciences, policy evaluation, and market research.
- Quasi-experimental research can be more practical and ethical compared to true experiments.
- While they offer flexibility, these designs require careful consideration of confounding variables.
Understanding Quasi-Experimental Research Design
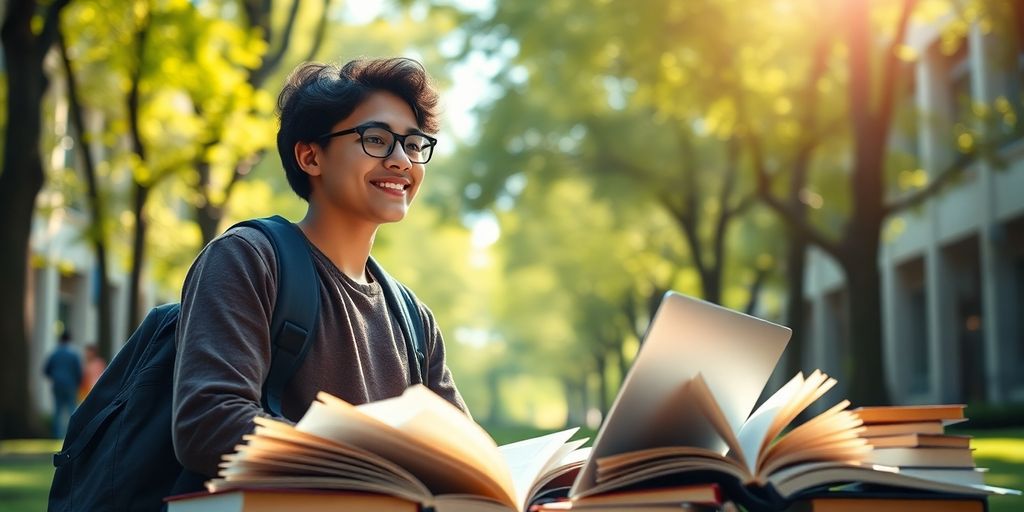
Defining Quasi-Experimental Design
A quasi-experimental design is a research method that shares similarities with true experimental design but differs in one critical aspect: the lack of random assignment. This approach is often chosen when it's impractical or unethical to randomly assign participants to different groups. In such studies, researchers manipulate an independent variable to observe its effect on a dependent variable, despite not having full control over the experimental conditions. This lack of randomization can lead to challenges in confidently attributing causality, yet it allows for real-world applications that might not be feasible otherwise.
Key Characteristics of Quasi-Experimental Research
Quasi-experimental research is marked by several defining traits:
- Absence of Randomization: Participants are not randomly assigned to treatment or control groups, often relying on naturally occurring groups instead.
- Manipulation of Variables: Despite the absence of randomization, researchers still intervene by introducing a treatment condition, aiming to measure its impact.
- Comparison Groups: Typically involves a treatment group and a control group, though these are not formed through random assignment.
- Mitigation of Confounding Variables: Researchers need to address potential confounding variables that might influence the outcome.
Distinguishing Features from True Experimental Design
While both true experimental and quasi-experimental designs involve manipulation of variables, the key difference lies in the assignment of participants. True experiments use random assignment, providing greater internal validity and stronger causal inferences. In contrast, quasi-experiments lack this randomization, which can introduce bias but offers greater external validity due to their application in more natural settings. This makes them particularly useful in fields like social science and policy evaluation, where choosing the right study design is crucial for effective research outcomes. Despite their limitations, quasi-experimental designs remain a valuable tool for researchers seeking to explore causal relationships in complex, real-world environments, much like how to write your thesis in 30 days requires a structured and adaptable approach.
Applications of Quasi-Experimental Design in Real-World Research
Quasi-experimental designs are a staple in social science research, allowing researchers to explore causal relationships in settings where traditional experiments aren't feasible. These designs are invaluable when ethical or practical constraints prevent random assignment. For example, when studying the impact of educational interventions, researchers might use existing class assignments as groups, comparing outcomes between those exposed to a new teaching method and those who are not. This approach can reveal significant insights into educational practices and policy impacts.
Implementing Quasi-Experimental Methods in Policy Evaluation
In policy evaluation, quasi-experimental designs help assess the effectiveness of government programs and initiatives. They enable the examination of outcomes before and after policy implementation, using methods like interrupted time-series analysis to identify changes attributable to the policy. This can be particularly useful in evaluating public health interventions, where randomization may not be possible. By comparing regions with and without the policy, researchers can infer the policy's impact, providing data-driven insights for future decision-making.
Market Research and Quasi-Experimental Approaches
Market researchers often turn to quasi-experimental designs to evaluate the success of marketing strategies and consumer behavior. For instance, when introducing a new product, businesses might compare sales trends in areas where the product is launched against those where it's not. Similarly, non-experimental research methods can be used to assess the impact of advertising campaigns by analyzing changes in brand awareness or sales. This method allows firms to gauge the effectiveness of their strategies without the need for costly and time-consuming randomized trials. By leveraging existing data and naturally occurring groups, companies can make informed decisions that directly influence their marketing tactics.
Types of Quasi-Experimental Designs
When diving into the realm of quasi-experimental research, it's essential to understand the different types of designs available. Each type offers unique ways to tackle research questions, especially when random assignment is not feasible. Let's explore some of the main quasi-experimental designs.
Nonequivalent Groups Design
The nonequivalent groups design is one of the most common forms used in quasi-experimental research. In this setup, researchers compare outcomes between groups that have not been randomly assigned. Imagine you want to study the effectiveness of a new teaching method. You might have one class use the new method while another class continues with the traditional approach. Both groups are given a pretest and a posttest to measure the impact. The key here is that the groups are as similar as possible, but without random assignment, they might still differ in ways that could affect the results.
Regression Discontinuity Design
Regression discontinuity design is a bit more complex but offers a clever way to assess causal effects. It involves assigning participants to different groups based on a cutoff score on a pre-treatment variable. For example, students might receive additional tutoring if their test scores fall below a certain threshold. By comparing those just above and below the cutoff, researchers can estimate the treatment's effect. This design helps in situations where ethical or practical reasons prevent random assignment.
Interrupted Time Series Design
The interrupted time series design is all about tracking changes over time. Researchers collect data at multiple intervals before and after an intervention. Let's say a new law is implemented to reduce traffic accidents. By analyzing accident rates before and after the law's enactment, researchers can infer its impact. This design is powerful for assessing long-term effects, though it requires a substantial amount of data and careful consideration of external factors that might influence the results.
In conclusion, understanding these designs is crucial for anyone conducting quasi-experimental studies. They provide flexibility in real-world settings where traditional experimental methods might not be applicable. Each design has its strengths and limitations, making it vital to choose the one that best fits your research context.
Advantages and Limitations of Quasi-Experimental Research
Benefits of Quasi-Experimental Design
Quasi-experimental research is like a Swiss Army knife for researchers. It's super handy when you can't use a true experimental design. One of its biggest perks is how well it fits into real-world scenarios, especially when dealing with social issues, policies, or market trends. Imagine trying to test the impact of WhatsApp on communication habits without being able to randomly assign people to use it or not. That's where quasi-experiments shine.
Here are a few reasons why researchers love this approach:
- Real-world applicability: You can study events or phenomena as they naturally occur, like policy changes or natural disasters, without needing to manipulate the environment artificially.
- Higher external validity: Since these studies often happen in natural settings, the findings tend to be more relatable to the real world.
- Cost-effective: Quasi-experiments often use existing data or naturally occurring groups, saving time and money compared to setting up a full-blown experiment.
Challenges and Limitations
But it's not all sunshine and rainbows. Quasi-experimental designs come with their own set of challenges. The biggest headache? Lower internal validity. Without random assignment, it's tough to rule out other reasons for your results. Maybe the people using WhatsApp were already better at communicating.
Here's what researchers have to watch out for:
- Selection bias: Pre-existing differences between groups can mess with the results. It's like comparing apples to oranges.
- Confounding variables: These pesky variables can sneak in and affect the outcome, making it hard to pinpoint what's really going on.
- Regression to the mean: Especially in pretest-posttest setups, this can lead to wrong conclusions about what's causing changes.
Balancing Internal and External Validity
Finding the sweet spot between internal and external validity is a balancing act. Internal validity is all about making sure your results are due to the treatment, while external validity is about how well those results apply outside the study.
In quasi-experimental research, you often have to trade a bit of internal validity for the sake of external validity. It's like trying to balance on a seesaw; you can't have both sides perfectly level. But with careful planning and statistical techniques, researchers can still draw meaningful conclusions.
In the end, quasi-experimental designs are a practical choice when randomization isn't possible. They allow for meaningful insights into real-world phenomena, like how WhatsApp influences communication patterns, despite their limitations.
Methodological Considerations in Quasi-Experimental Research
Addressing Confounding Variables
When diving into quasi-experimental research, one of the first hurdles is dealing with confounding variables. These are the pesky factors that can skew results if not handled properly. Unlike true experiments, where random assignment can help balance these out, quasi-experiments have to get creative. Researchers often use techniques like matching or statistical controls to level the playing field. Imagine you're studying the impact of a new teaching method in schools. If one school has newer facilities, that could influence the results. Adjusting for such variables is key to making sure the findings are valid.
Ensuring Validity and Reliability
Ensuring that your research is both valid and reliable is like making sure your Instagram feed is both trendy and consistent. Validity means you're actually measuring what you think you're measuring. Reliability is about getting the same results if you did the study again. In quasi-experimental designs, this can be tricky. Researchers often rely on repeated measures or triangulation, using multiple methods or data sources to confirm findings. This way, they can be more confident that their results are not just a fluke.
Ethical Considerations in Design
Ethical concerns are always a hot topic, and in quasi-experimental research, they can be quite the balancing act. Since random assignment isn't used, researchers must be extra careful about how participants are selected and treated. It's crucial to ensure that no group is unfairly advantaged or disadvantaged by the study. For instance, if a study involves a new health intervention, researchers must ensure that those not receiving the intervention aren't at risk. Transparency with participants about the nature of the study and its potential impacts is also a must.
Case Studies and Examples of Quasi-Experimental Design
In education, quasi-experimental designs are often used to evaluate the impact of new teaching methods or educational programs. For instance, a school district might introduce a new curriculum in select schools while maintaining the existing one in others. This setup allows researchers to compare student performance across different teaching methods without random assignment. Such studies can reveal insights into educational practices that might be more effective for certain student populations.
Public Health Applications
Quasi-experimental designs are also prevalent in public health research. They help assess the effects of health interventions when randomized trials aren't feasible due to ethical or logistical reasons. For example, a city might implement a smoking cessation program in certain neighborhoods and use others as a control. Researchers can then compare health outcomes like smoking rates or respiratory health between these areas, offering valuable data on the program's effectiveness.
Market Research Case Studies
In the realm of market research, quasi-experimental designs offer a practical way to test hypotheses about consumer behavior. Consider a retail chain assessing a new store layout's impact on sales. Stores with the new layout serve as the experimental group, while those with the old design act as the control. Over time, sales data from both groups are analyzed to determine the layout's effectiveness. Another example includes an online retailer using a regression discontinuity design to evaluate a loyalty program by comparing purchasing behavior just above and below a spending threshold. These approaches allow businesses to make informed decisions based on real-world data.
For those interested in exploring past events to understand their impacts, ex post facto research provides a method to analyze relationships between variables through observational data, offering insights into policy development and behavioral influences.
Statistical Techniques in Quasi-Experimental Research
Quasi-experimental research is a fascinating field, offering a middle ground between true experiments and observational studies. While it lacks the rigor of random assignment, it compensates with real-world applicability. To make the most of this design, researchers often rely on a variety of statistical techniques. Here, we'll explore some of the most common methods used to analyze quasi-experimental data.
Propensity Score Matching
Propensity score matching is a statistical method used to create a balanced comparison between groups in a study. This technique attempts to mimic random assignment by matching participants based on similar characteristics. By doing so, it helps control for confounding variables and reduces bias, making it easier to identify the treatment effect. This method is particularly useful in studies where randomization isn't feasible.
Regression Analysis in Quasi-Experiments
Regression analysis is another powerful tool in the arsenal of quasi-experimental research. It allows researchers to assess the relationship between variables and control for potential confounders. In this context, multiple regression can be used to adjust for various factors that might influence the outcome. By incorporating these variables into the model, researchers can better isolate the effect of the treatment.
Handling Missing Data
Missing data is a common issue in any research, and quasi-experimental studies are no exception. Techniques such as multiple imputation and maximum likelihood estimation are often employed to address this problem. These methods help to fill in the gaps, ensuring that the analysis remains robust and reliable. Handling missing data properly is crucial to maintaining the integrity of the study's findings.
In summary, while quasi-experimental designs may not offer the same level of control as true experiments, they provide valuable insights into causal relationships in real-world settings. By employing statistical techniques like propensity score matching, regression analysis, and methods for handling missing data, researchers can enhance the validity and reliability of their findings.
In quasi-experimental research, statistical methods play a key role in understanding data. These techniques help researchers make sense of their findings and draw meaningful conclusions. If you're a student feeling overwhelmed by your thesis, don't worry! Visit our website to discover how our Thesis Action Plan can guide you through every step of the writing process. Take control of your academic journey today!
Conclusion
In wrapping up our exploration of quasi-experimental research design, it's clear that this approach offers a practical solution for real-world research challenges. While it doesn't boast the same level of control as true experimental designs, its flexibility and applicability in natural settings make it invaluable. By allowing researchers to study causal relationships without the constraints of randomization, quasi-experimental designs open doors to insights that might otherwise remain unexplored. Whether in social sciences, policy evaluation, or market research, this method provides a robust framework for understanding complex phenomena. As we continue to refine these techniques, the potential for meaningful discoveries only grows. Embracing the strengths and acknowledging the limitations of quasi-experimental designs will ensure they remain a vital tool in the researcher's toolkit.
Frequently Asked Questions
What exactly is a quasi-experimental design?
A quasi-experimental design is a research method that looks a lot like a real experiment but doesn't use random assignment. Researchers study how one thing affects another in real-life settings without randomly choosing who gets what treatment.
How does a quasi-experimental design differ from a true experiment?
The big difference is in how participants are assigned to groups. In true experiments, participants are randomly assigned to groups, but in quasi-experiments, they aren't. This means quasi-experiments might have more biases, but they can still be useful.
Why would someone choose a quasi-experimental design over a true experiment?
Sometimes it's not possible or ethical to randomly assign people to groups, like when studying the effects of a new teaching method in schools. Quasi-experimental designs let researchers study real-world situations without needing random assignment.
Can you give an example of a quasi-experimental design?
Sure! Imagine a study that looks at how a new reading program affects students' test scores. Instead of randomly assigning students to use the new program, researchers might compare scores from two existing classes, one using the program and one not.
What are the advantages of using a quasi-experimental design?
Quasi-experimental designs are great for studying real-world situations where random assignment isn't possible. They offer more flexibility and can be less costly and time-consuming than true experiments.
Are there any downsides to quasi-experimental designs?
Yes, because they don't use random assignment, they might have more biases and less control over variables. This can make it harder to be sure that the treatment caused the effects seen in the study.
Master Quasi-Experimental Research Design for Real-World Applications

So, you're diving into the world of quasi-experimental research design, huh? It's one of those things that sounds super complicated, but once you get the hang of it, it can be a game-changer. This type of research is like a middle ground between full-blown experiments and just observing stuff. You don't get to randomly assign people or things to groups, which can be a bit of a bummer, but it's perfect for real-world situations where you can't just shuffle things around like a deck of cards. You still get to play around with variables and see what happens, which is pretty cool.
Key Takeaways
- Quasi-experimental designs are great for real-world research where random assignments aren't possible.
- These designs help in studying cause-and-effect relationships without the strict controls of true experiments.
- They are widely used in fields like social sciences, policy evaluation, and market research.
- Quasi-experimental research can be more practical and ethical compared to true experiments.
- While they offer flexibility, these designs require careful consideration of confounding variables.
Understanding Quasi-Experimental Research Design
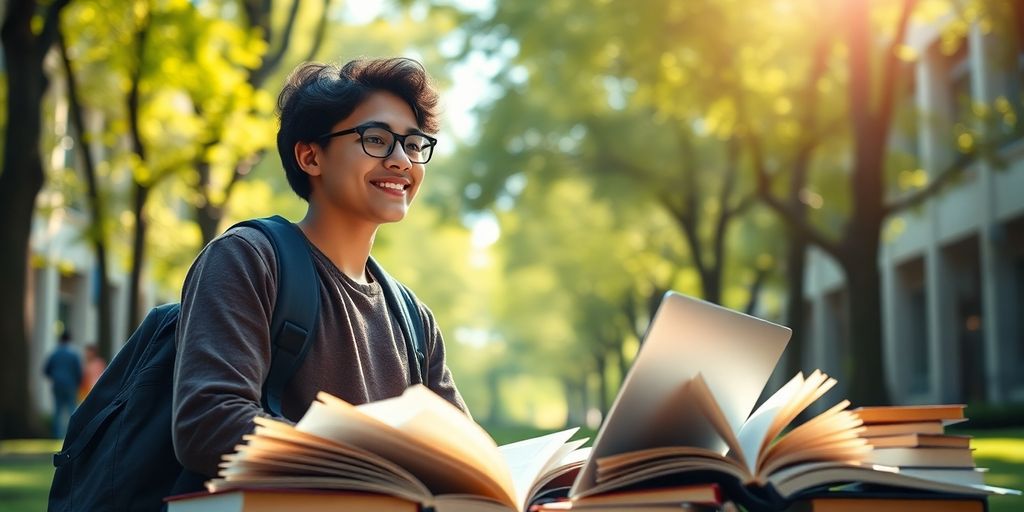
Defining Quasi-Experimental Design
A quasi-experimental design is a research method that shares similarities with true experimental design but differs in one critical aspect: the lack of random assignment. This approach is often chosen when it's impractical or unethical to randomly assign participants to different groups. In such studies, researchers manipulate an independent variable to observe its effect on a dependent variable, despite not having full control over the experimental conditions. This lack of randomization can lead to challenges in confidently attributing causality, yet it allows for real-world applications that might not be feasible otherwise.
Key Characteristics of Quasi-Experimental Research
Quasi-experimental research is marked by several defining traits:
- Absence of Randomization: Participants are not randomly assigned to treatment or control groups, often relying on naturally occurring groups instead.
- Manipulation of Variables: Despite the absence of randomization, researchers still intervene by introducing a treatment condition, aiming to measure its impact.
- Comparison Groups: Typically involves a treatment group and a control group, though these are not formed through random assignment.
- Mitigation of Confounding Variables: Researchers need to address potential confounding variables that might influence the outcome.
Distinguishing Features from True Experimental Design
While both true experimental and quasi-experimental designs involve manipulation of variables, the key difference lies in the assignment of participants. True experiments use random assignment, providing greater internal validity and stronger causal inferences. In contrast, quasi-experiments lack this randomization, which can introduce bias but offers greater external validity due to their application in more natural settings. This makes them particularly useful in fields like social science and policy evaluation, where choosing the right study design is crucial for effective research outcomes. Despite their limitations, quasi-experimental designs remain a valuable tool for researchers seeking to explore causal relationships in complex, real-world environments, much like how to write your thesis in 30 days requires a structured and adaptable approach.
Applications of Quasi-Experimental Design in Real-World Research
Quasi-experimental designs are a staple in social science research, allowing researchers to explore causal relationships in settings where traditional experiments aren't feasible. These designs are invaluable when ethical or practical constraints prevent random assignment. For example, when studying the impact of educational interventions, researchers might use existing class assignments as groups, comparing outcomes between those exposed to a new teaching method and those who are not. This approach can reveal significant insights into educational practices and policy impacts.
Implementing Quasi-Experimental Methods in Policy Evaluation
In policy evaluation, quasi-experimental designs help assess the effectiveness of government programs and initiatives. They enable the examination of outcomes before and after policy implementation, using methods like interrupted time-series analysis to identify changes attributable to the policy. This can be particularly useful in evaluating public health interventions, where randomization may not be possible. By comparing regions with and without the policy, researchers can infer the policy's impact, providing data-driven insights for future decision-making.
Market Research and Quasi-Experimental Approaches
Market researchers often turn to quasi-experimental designs to evaluate the success of marketing strategies and consumer behavior. For instance, when introducing a new product, businesses might compare sales trends in areas where the product is launched against those where it's not. Similarly, non-experimental research methods can be used to assess the impact of advertising campaigns by analyzing changes in brand awareness or sales. This method allows firms to gauge the effectiveness of their strategies without the need for costly and time-consuming randomized trials. By leveraging existing data and naturally occurring groups, companies can make informed decisions that directly influence their marketing tactics.
Types of Quasi-Experimental Designs
When diving into the realm of quasi-experimental research, it's essential to understand the different types of designs available. Each type offers unique ways to tackle research questions, especially when random assignment is not feasible. Let's explore some of the main quasi-experimental designs.
Nonequivalent Groups Design
The nonequivalent groups design is one of the most common forms used in quasi-experimental research. In this setup, researchers compare outcomes between groups that have not been randomly assigned. Imagine you want to study the effectiveness of a new teaching method. You might have one class use the new method while another class continues with the traditional approach. Both groups are given a pretest and a posttest to measure the impact. The key here is that the groups are as similar as possible, but without random assignment, they might still differ in ways that could affect the results.
Regression Discontinuity Design
Regression discontinuity design is a bit more complex but offers a clever way to assess causal effects. It involves assigning participants to different groups based on a cutoff score on a pre-treatment variable. For example, students might receive additional tutoring if their test scores fall below a certain threshold. By comparing those just above and below the cutoff, researchers can estimate the treatment's effect. This design helps in situations where ethical or practical reasons prevent random assignment.
Interrupted Time Series Design
The interrupted time series design is all about tracking changes over time. Researchers collect data at multiple intervals before and after an intervention. Let's say a new law is implemented to reduce traffic accidents. By analyzing accident rates before and after the law's enactment, researchers can infer its impact. This design is powerful for assessing long-term effects, though it requires a substantial amount of data and careful consideration of external factors that might influence the results.
In conclusion, understanding these designs is crucial for anyone conducting quasi-experimental studies. They provide flexibility in real-world settings where traditional experimental methods might not be applicable. Each design has its strengths and limitations, making it vital to choose the one that best fits your research context.
Advantages and Limitations of Quasi-Experimental Research
Benefits of Quasi-Experimental Design
Quasi-experimental research is like a Swiss Army knife for researchers. It's super handy when you can't use a true experimental design. One of its biggest perks is how well it fits into real-world scenarios, especially when dealing with social issues, policies, or market trends. Imagine trying to test the impact of WhatsApp on communication habits without being able to randomly assign people to use it or not. That's where quasi-experiments shine.
Here are a few reasons why researchers love this approach:
- Real-world applicability: You can study events or phenomena as they naturally occur, like policy changes or natural disasters, without needing to manipulate the environment artificially.
- Higher external validity: Since these studies often happen in natural settings, the findings tend to be more relatable to the real world.
- Cost-effective: Quasi-experiments often use existing data or naturally occurring groups, saving time and money compared to setting up a full-blown experiment.
Challenges and Limitations
But it's not all sunshine and rainbows. Quasi-experimental designs come with their own set of challenges. The biggest headache? Lower internal validity. Without random assignment, it's tough to rule out other reasons for your results. Maybe the people using WhatsApp were already better at communicating.
Here's what researchers have to watch out for:
- Selection bias: Pre-existing differences between groups can mess with the results. It's like comparing apples to oranges.
- Confounding variables: These pesky variables can sneak in and affect the outcome, making it hard to pinpoint what's really going on.
- Regression to the mean: Especially in pretest-posttest setups, this can lead to wrong conclusions about what's causing changes.
Balancing Internal and External Validity
Finding the sweet spot between internal and external validity is a balancing act. Internal validity is all about making sure your results are due to the treatment, while external validity is about how well those results apply outside the study.
In quasi-experimental research, you often have to trade a bit of internal validity for the sake of external validity. It's like trying to balance on a seesaw; you can't have both sides perfectly level. But with careful planning and statistical techniques, researchers can still draw meaningful conclusions.
In the end, quasi-experimental designs are a practical choice when randomization isn't possible. They allow for meaningful insights into real-world phenomena, like how WhatsApp influences communication patterns, despite their limitations.
Methodological Considerations in Quasi-Experimental Research
Addressing Confounding Variables
When diving into quasi-experimental research, one of the first hurdles is dealing with confounding variables. These are the pesky factors that can skew results if not handled properly. Unlike true experiments, where random assignment can help balance these out, quasi-experiments have to get creative. Researchers often use techniques like matching or statistical controls to level the playing field. Imagine you're studying the impact of a new teaching method in schools. If one school has newer facilities, that could influence the results. Adjusting for such variables is key to making sure the findings are valid.
Ensuring Validity and Reliability
Ensuring that your research is both valid and reliable is like making sure your Instagram feed is both trendy and consistent. Validity means you're actually measuring what you think you're measuring. Reliability is about getting the same results if you did the study again. In quasi-experimental designs, this can be tricky. Researchers often rely on repeated measures or triangulation, using multiple methods or data sources to confirm findings. This way, they can be more confident that their results are not just a fluke.
Ethical Considerations in Design
Ethical concerns are always a hot topic, and in quasi-experimental research, they can be quite the balancing act. Since random assignment isn't used, researchers must be extra careful about how participants are selected and treated. It's crucial to ensure that no group is unfairly advantaged or disadvantaged by the study. For instance, if a study involves a new health intervention, researchers must ensure that those not receiving the intervention aren't at risk. Transparency with participants about the nature of the study and its potential impacts is also a must.
Case Studies and Examples of Quasi-Experimental Design
In education, quasi-experimental designs are often used to evaluate the impact of new teaching methods or educational programs. For instance, a school district might introduce a new curriculum in select schools while maintaining the existing one in others. This setup allows researchers to compare student performance across different teaching methods without random assignment. Such studies can reveal insights into educational practices that might be more effective for certain student populations.
Public Health Applications
Quasi-experimental designs are also prevalent in public health research. They help assess the effects of health interventions when randomized trials aren't feasible due to ethical or logistical reasons. For example, a city might implement a smoking cessation program in certain neighborhoods and use others as a control. Researchers can then compare health outcomes like smoking rates or respiratory health between these areas, offering valuable data on the program's effectiveness.
Market Research Case Studies
In the realm of market research, quasi-experimental designs offer a practical way to test hypotheses about consumer behavior. Consider a retail chain assessing a new store layout's impact on sales. Stores with the new layout serve as the experimental group, while those with the old design act as the control. Over time, sales data from both groups are analyzed to determine the layout's effectiveness. Another example includes an online retailer using a regression discontinuity design to evaluate a loyalty program by comparing purchasing behavior just above and below a spending threshold. These approaches allow businesses to make informed decisions based on real-world data.
For those interested in exploring past events to understand their impacts, ex post facto research provides a method to analyze relationships between variables through observational data, offering insights into policy development and behavioral influences.
Statistical Techniques in Quasi-Experimental Research
Quasi-experimental research is a fascinating field, offering a middle ground between true experiments and observational studies. While it lacks the rigor of random assignment, it compensates with real-world applicability. To make the most of this design, researchers often rely on a variety of statistical techniques. Here, we'll explore some of the most common methods used to analyze quasi-experimental data.
Propensity Score Matching
Propensity score matching is a statistical method used to create a balanced comparison between groups in a study. This technique attempts to mimic random assignment by matching participants based on similar characteristics. By doing so, it helps control for confounding variables and reduces bias, making it easier to identify the treatment effect. This method is particularly useful in studies where randomization isn't feasible.
Regression Analysis in Quasi-Experiments
Regression analysis is another powerful tool in the arsenal of quasi-experimental research. It allows researchers to assess the relationship between variables and control for potential confounders. In this context, multiple regression can be used to adjust for various factors that might influence the outcome. By incorporating these variables into the model, researchers can better isolate the effect of the treatment.
Handling Missing Data
Missing data is a common issue in any research, and quasi-experimental studies are no exception. Techniques such as multiple imputation and maximum likelihood estimation are often employed to address this problem. These methods help to fill in the gaps, ensuring that the analysis remains robust and reliable. Handling missing data properly is crucial to maintaining the integrity of the study's findings.
In summary, while quasi-experimental designs may not offer the same level of control as true experiments, they provide valuable insights into causal relationships in real-world settings. By employing statistical techniques like propensity score matching, regression analysis, and methods for handling missing data, researchers can enhance the validity and reliability of their findings.
In quasi-experimental research, statistical methods play a key role in understanding data. These techniques help researchers make sense of their findings and draw meaningful conclusions. If you're a student feeling overwhelmed by your thesis, don't worry! Visit our website to discover how our Thesis Action Plan can guide you through every step of the writing process. Take control of your academic journey today!
Conclusion
In wrapping up our exploration of quasi-experimental research design, it's clear that this approach offers a practical solution for real-world research challenges. While it doesn't boast the same level of control as true experimental designs, its flexibility and applicability in natural settings make it invaluable. By allowing researchers to study causal relationships without the constraints of randomization, quasi-experimental designs open doors to insights that might otherwise remain unexplored. Whether in social sciences, policy evaluation, or market research, this method provides a robust framework for understanding complex phenomena. As we continue to refine these techniques, the potential for meaningful discoveries only grows. Embracing the strengths and acknowledging the limitations of quasi-experimental designs will ensure they remain a vital tool in the researcher's toolkit.
Frequently Asked Questions
What exactly is a quasi-experimental design?
A quasi-experimental design is a research method that looks a lot like a real experiment but doesn't use random assignment. Researchers study how one thing affects another in real-life settings without randomly choosing who gets what treatment.
How does a quasi-experimental design differ from a true experiment?
The big difference is in how participants are assigned to groups. In true experiments, participants are randomly assigned to groups, but in quasi-experiments, they aren't. This means quasi-experiments might have more biases, but they can still be useful.
Why would someone choose a quasi-experimental design over a true experiment?
Sometimes it's not possible or ethical to randomly assign people to groups, like when studying the effects of a new teaching method in schools. Quasi-experimental designs let researchers study real-world situations without needing random assignment.
Can you give an example of a quasi-experimental design?
Sure! Imagine a study that looks at how a new reading program affects students' test scores. Instead of randomly assigning students to use the new program, researchers might compare scores from two existing classes, one using the program and one not.
What are the advantages of using a quasi-experimental design?
Quasi-experimental designs are great for studying real-world situations where random assignment isn't possible. They offer more flexibility and can be less costly and time-consuming than true experiments.
Are there any downsides to quasi-experimental designs?
Yes, because they don't use random assignment, they might have more biases and less control over variables. This can make it harder to be sure that the treatment caused the effects seen in the study.