The Ultimate Guide to Designing Causal Research That Gets Results

So you're thinking about diving into causal research design, huh? It's like figuring out a puzzle, but with science. This guide is all about helping you piece it together. Whether you're a newbie or a seasoned pro, understanding the basics and getting the right tools is key. From setting your research questions to tackling ethical dilemmas, we've got it all covered. Let's make sure your research doesn't just sit on a shelf but actually gets results.
Key Takeaways
- Get clear on what causal research design really means and why it matters.
- Crafting solid research questions and hypotheses is the backbone of any study.
- Picking the right research design type can make or break your project.
- Collecting and analyzing data is where the magic happens, but it needs to be done right.
- Don't ignore the ethical and practical challenges; they can derail your research if not handled.
Understanding the Foundations of Causal Research Design
Defining Causal Research Design
Causal research design is all about figuring out the cause-and-effect relationships between variables. Imagine you're trying to see if drinking coffee makes people more alert. That's what causal research aims to do—determine if one thing causes another. It's a big deal because it helps in making predictions and understanding the world better. Causal research design is crucial because it allows researchers to make informed decisions based on evidence. Unlike correlational studies, which only show that two things are related, causal research digs deeper to find out why and how they're connected.
Historical Context and Evolution
The journey of causal research design is pretty fascinating. It started centuries ago when scholars began questioning the "why" behind natural phenomena. Over time, this curiosity evolved into structured methods for testing hypotheses. In the last century, the development of experimental and quasi-experimental designs has been pivotal in refining these methods. Today, causal research is more sophisticated, thanks to technological advancements and effective research design principles. These developments have made it easier to replicate studies and ensure that findings are reliable and valid.
Key Principles and Concepts
At the heart of causal research design are some key principles. First, there's the idea of manipulation—changing one variable to see what happens to another. Next is control, where researchers try to keep everything else constant to ensure that any changes in the outcome are due to the manipulated variable. Randomization is another biggie, helping to minimize biases and ensure that results are not skewed by other factors. These principles are essential for maintaining the integrity of the research and ensuring that the results are trustworthy.
In summary, understanding the foundations of causal research design is about knowing how to set up a study that can truly test cause-and-effect relationships. It's about being meticulous and thoughtful in every step, from defining the research question to choosing the right method. With a solid grasp of these concepts, researchers can produce findings that are not only insightful but also reliable and applicable in real-world scenarios.
Formulating Research Questions and Hypotheses
Crafting Precise Research Questions
Crafting precise research questions is the cornerstone of any successful study. These questions guide the entire research process, helping to focus efforts and resources on the most relevant aspects. To formulate a research question, start by identifying the problem or topic of interest. Break it down into smaller, manageable parts to clarify what you need to investigate. This process is similar to how one might approach AI's value creation, where philosophical frameworks guide learning processes. Consider the following steps:
- Identify the broad topic or issue you want to explore.
- Narrow it down to specific aspects that are feasible to study.
- Frame your question in a way that is clear, focused, and researchable.
Developing Testable Hypotheses
Once you have a clear research question, the next step is to develop a hypothesis. A hypothesis is a testable statement that predicts the relationship between variables. It should stem from your research question and be informed by existing literature. For example, if your research question is about the impact of social media on job search success, you might hypothesize that "LinkedIn usage increases job acquisition rates." Here’s how to write your hypothesis:
- Clearly state the expected relationship between variables.
- Ensure it is testable with the available methods.
- Align it with the research design and objectives.
Aligning Questions with Research Objectives
Aligning your research questions with your objectives ensures that your study remains focused and relevant. This alignment helps in maintaining a clear trajectory throughout your research process. To achieve this, regularly revisit your objectives to see if your questions still support them. This is especially important if you’re working on a tight timeline, like when figuring out how to write your thesis in 30 days. Keep these points in mind:
- Regularly review and adjust your questions to fit your objectives.
- Ensure that each question contributes to achieving your overall goals.
- Use your objectives as a checklist to evaluate the relevance of your questions.
Selecting Appropriate Research Design Types
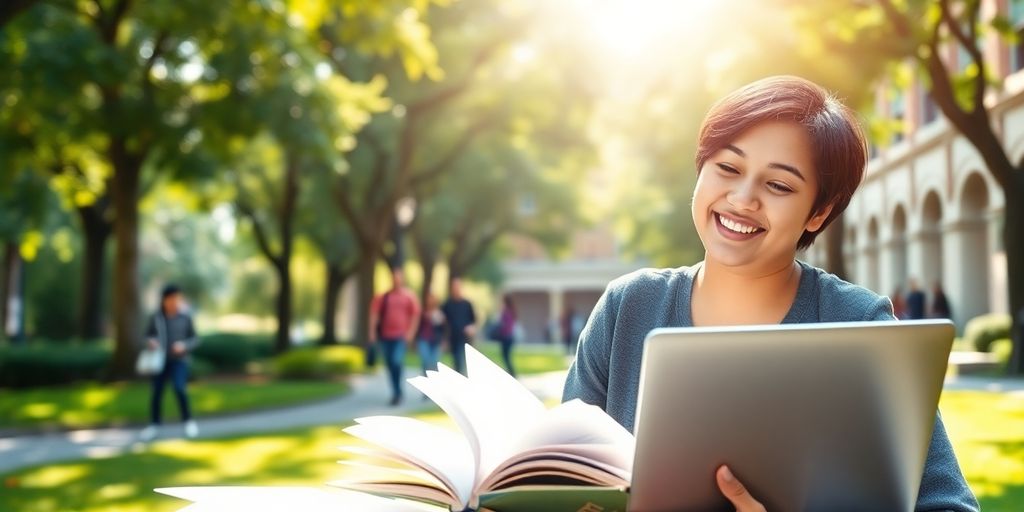
Choosing the right research design is like picking the perfect tool for a job. It’s all about matching the design to your specific research questions and goals. Here, we’ll dive into the main types of research designs you might consider.
Experimental vs. Non-Experimental Designs
When it comes to research, you’ve got two main roads: experimental and non-experimental. Experimental designs are all about control and manipulation. You set up experiments to test cause-and-effect relationships by manipulating variables and observing the outcomes. Think of it like a science lab where you’re the one conducting the tests. On the other hand, non-experimental designs are more observational. You’re not changing anything, just watching and recording what happens naturally. This can be useful when manipulation isn’t possible or ethical.
Quasi-Experimental Design Considerations
Quasi-experimental designs sit somewhere in the middle. They’re like experimental designs but without the full control over variables. This might happen if you can’t randomly assign participants to groups. For example, you might study the effect of a new teaching method in two different schools, but you can’t control which students go to which school. It’s a bit of a compromise, but it allows for real-world application while still trying to infer causality.
Choosing the Right Design for Your Study
Picking the right design depends on a few things:
- Research Question: What are you trying to find out? If you’re looking at cause and effect, experimental might be your best bet.
- Resources: Do you have the time and money for a full-blown experiment? If not, non-experimental might be the way to go.
- Ethics: Sometimes, you just can’t manipulate variables because it wouldn’t be right. In these cases, non-experimental or quasi-experimental designs are more appropriate.
By understanding these options, you can make an informed choice that aligns with your research goals. For more insights on aligning research design with your objectives, check out this guide that covers various types of quantitative research designs and their applications.
Data Collection and Analysis in Causal Research
Methods for Collecting Reliable Data
In causal research, gathering data is a crucial step. You need to decide how to collect data, whether through surveys, interviews, or even digital means like WhatsApp. Each method has its pros and cons, so think about what fits your study best. Surveys can reach a large audience, but interviews might give you deeper insights. Digital tools can offer convenience and real-time data but might miss out on personal nuances.
Analyzing Data for Causal Inference
Once you've gathered your data, the next step is analysis. This is where you determine if there's a causal link between variables. Statistical software can help, but it's important to choose the right tests. For example, regression analysis might be suitable for some studies, while others might require more complex models. The key is to ensure your analysis aligns with your research questions.
Ensuring Data Validity and Reliability
Finally, it's essential to make sure your data is both valid and reliable. Validity means your data accurately reflects what you aim to measure. Reliability means you could get the same results if you repeated the study. To achieve this, consider techniques like triangulation, where you use multiple methods to collect data. This can help identify any inconsistencies and strengthen your findings.
Addressing Ethical and Practical Challenges
Ethical Considerations in Causal Research
When designing causal research, ethical considerations are a key concern. Researchers must prioritize obtaining informed consent from participants, ensuring that they are fully aware of the study's nature and their role in it. Confidentiality is another critical aspect, requiring researchers to safeguard participants' personal information. Additionally, any potential harm or discomfort to participants should be minimized. In the context of ex post facto design research, ethical challenges also arise from analyzing pre-existing data, where issues of privacy and consent can become complex.
Overcoming Practical Limitations
Practical limitations often present significant hurdles in causal research design. These can include limited resources, time constraints, and difficulties in accessing data or participants. Researchers must navigate these challenges by making strategic choices, such as prioritizing certain aspects of the study over others or seeking alternative data sources. A well-thought-out plan can help ensure that the research remains feasible and maintains its integrity. In some cases, leveraging technology or collaborating with other researchers can also provide creative solutions to practical obstacles.
Balancing Scientific Rigor with Feasibility
Achieving a balance between scientific rigor and feasibility is crucial in causal research. While it is important to maintain a high standard of scientific accuracy, researchers must also consider the practical aspects of conducting their studies. This might mean adapting methodologies to fit within budgetary constraints or modifying timelines to accommodate unexpected delays. The ethics review process, especially for internet-based research, often requires researchers to adapt their designs to meet both ethical standards and practical realities. By carefully planning and remaining flexible, researchers can conduct studies that are both ethically sound and practically viable.
Enhancing Research Design with Advanced Tools
Utilizing Technology in Research Design
In today's research landscape, technology plays a key role in shaping how studies are conducted. From data collection to analysis, tech tools streamline processes and make complex tasks more manageable. Tech advancements allow researchers to collect high-quality data efficiently, making it easier to draw meaningful conclusions. For instance, social media platforms like Instagram can be used to gather data on user behavior and preferences, offering insights that were previously hard to obtain.
Incorporating Biosensors and iMotions
Biosensors, like eye trackers and EEG devices, provide researchers with the ability to capture data that goes beyond traditional methods. These tools offer a window into the physiological and emotional states of participants, enabling a deeper understanding of human behavior. iMotions software, in particular, is a powerful tool for integrating data from multiple biosensors, providing a comprehensive view of participant responses. This integration is particularly useful in studies where understanding the interaction between different stimuli and responses is crucial.
Leveraging Software for Data Integration
The use of advanced software solutions is pivotal in handling and integrating large datasets. These tools help in organizing data efficiently, ensuring that researchers can focus on analysis rather than data management. Software solutions provide the framework for combining various data types, making it easier to interpret complex datasets. This is especially important in studies that require the synthesis of qualitative and quantitative data to form a cohesive understanding of the research questions.
Ensuring Validity and Reliability in Causal Research
Internal vs. External Validity
When you're diving into causal research, understanding the difference between internal and external validity is key. Internal validity asks if the study truly shows a cause-and-effect relationship between variables. Imagine you're testing if a new teaching method improves student grades. If your study is internally valid, you can confidently say that any grade changes are due to your method, not some other factor like extra tutoring. On the flip side, external validity is all about generalizability. Can your findings be applied to other settings or populations? If you discover that your teaching method works wonders in one school, external validity checks if it would work just as well elsewhere.
Strategies for Enhancing Reliability
Reliability is about consistency. If you measure something today and again tomorrow, will you get the same result? That's reliability. To boost it, consider these strategies:
- Standardize Procedures: Keep your methods consistent across all participants and trials.
- Use Reliable Instruments: Ensure your tools and measures are tested and proven to give stable results.
- Train Researchers: Make sure everyone involved in data collection is on the same page and knows exactly what to do.
Replicability and Generalizability of Findings
Replicability is like the gold standard in research. If someone else can repeat your study and get the same results, that's a big win for scientific credibility. Generalizability, meanwhile, asks if your findings apply beyond your study's specific context. Say you conducted research on stress and productivity in a tech company. Can those results be applied to other industries or job roles? That's the big question of generalizability. It's crucial to design studies that not only answer your research questions but also hold up when tested by others or in different settings.
When doing causal research, it's super important to make sure your results are both valid and reliable. This means you need to check that your methods are solid and that your findings can be trusted. If you're feeling stuck or unsure about how to tackle your thesis, don't worry! Visit our website for helpful tips and a step-by-step plan to guide you through the process. Let's make your thesis journey easier together!
Conclusion
In wrapping up our exploration of designing causal research, it's clear that a well-thought-out approach is key to obtaining meaningful results. By carefully planning each step—from formulating research questions to selecting the right design and methods—researchers can ensure their studies are both effective and reliable. This guide has aimed to provide a practical framework for those embarking on this journey, emphasizing the importance of clarity and simplicity in research design. With these tools at your disposal, you're well-equipped to conduct research that not only answers your questions but also contributes valuable insights to your field. Remember, the success of your research lies in the details, so take the time to plan thoroughly and execute with precision.
Frequently Asked Questions
What is causal research design?
Causal research design is a way to find out if one thing causes another. It's like a detective solving a mystery by figuring out if one event leads to another.
Why is it important to have clear research questions?
Clear research questions help guide your study. They make sure you know exactly what you're trying to find out, so you don't get lost along the way.
How do you choose the right research design for a study?
Choosing the right research design depends on what you're trying to learn. You need to think about your goals, the questions you're asking, and the tools you have.
What are some methods to collect reliable data?
Reliable data can be collected using surveys, interviews, or experiments. It's important to choose a method that fits your research question and makes sense for your study.
What challenges might you face in causal research?
In causal research, you might face challenges like ethical issues, limited resources, or difficulty in controlling all the variables.
How can technology help in research design?
Technology can help by providing tools to collect and analyze data more efficiently. It makes the research process faster and often more accurate.
The Ultimate Guide to Designing Causal Research That Gets Results

So you're thinking about diving into causal research design, huh? It's like figuring out a puzzle, but with science. This guide is all about helping you piece it together. Whether you're a newbie or a seasoned pro, understanding the basics and getting the right tools is key. From setting your research questions to tackling ethical dilemmas, we've got it all covered. Let's make sure your research doesn't just sit on a shelf but actually gets results.
Key Takeaways
- Get clear on what causal research design really means and why it matters.
- Crafting solid research questions and hypotheses is the backbone of any study.
- Picking the right research design type can make or break your project.
- Collecting and analyzing data is where the magic happens, but it needs to be done right.
- Don't ignore the ethical and practical challenges; they can derail your research if not handled.
Understanding the Foundations of Causal Research Design
Defining Causal Research Design
Causal research design is all about figuring out the cause-and-effect relationships between variables. Imagine you're trying to see if drinking coffee makes people more alert. That's what causal research aims to do—determine if one thing causes another. It's a big deal because it helps in making predictions and understanding the world better. Causal research design is crucial because it allows researchers to make informed decisions based on evidence. Unlike correlational studies, which only show that two things are related, causal research digs deeper to find out why and how they're connected.
Historical Context and Evolution
The journey of causal research design is pretty fascinating. It started centuries ago when scholars began questioning the "why" behind natural phenomena. Over time, this curiosity evolved into structured methods for testing hypotheses. In the last century, the development of experimental and quasi-experimental designs has been pivotal in refining these methods. Today, causal research is more sophisticated, thanks to technological advancements and effective research design principles. These developments have made it easier to replicate studies and ensure that findings are reliable and valid.
Key Principles and Concepts
At the heart of causal research design are some key principles. First, there's the idea of manipulation—changing one variable to see what happens to another. Next is control, where researchers try to keep everything else constant to ensure that any changes in the outcome are due to the manipulated variable. Randomization is another biggie, helping to minimize biases and ensure that results are not skewed by other factors. These principles are essential for maintaining the integrity of the research and ensuring that the results are trustworthy.
In summary, understanding the foundations of causal research design is about knowing how to set up a study that can truly test cause-and-effect relationships. It's about being meticulous and thoughtful in every step, from defining the research question to choosing the right method. With a solid grasp of these concepts, researchers can produce findings that are not only insightful but also reliable and applicable in real-world scenarios.
Formulating Research Questions and Hypotheses
Crafting Precise Research Questions
Crafting precise research questions is the cornerstone of any successful study. These questions guide the entire research process, helping to focus efforts and resources on the most relevant aspects. To formulate a research question, start by identifying the problem or topic of interest. Break it down into smaller, manageable parts to clarify what you need to investigate. This process is similar to how one might approach AI's value creation, where philosophical frameworks guide learning processes. Consider the following steps:
- Identify the broad topic or issue you want to explore.
- Narrow it down to specific aspects that are feasible to study.
- Frame your question in a way that is clear, focused, and researchable.
Developing Testable Hypotheses
Once you have a clear research question, the next step is to develop a hypothesis. A hypothesis is a testable statement that predicts the relationship between variables. It should stem from your research question and be informed by existing literature. For example, if your research question is about the impact of social media on job search success, you might hypothesize that "LinkedIn usage increases job acquisition rates." Here’s how to write your hypothesis:
- Clearly state the expected relationship between variables.
- Ensure it is testable with the available methods.
- Align it with the research design and objectives.
Aligning Questions with Research Objectives
Aligning your research questions with your objectives ensures that your study remains focused and relevant. This alignment helps in maintaining a clear trajectory throughout your research process. To achieve this, regularly revisit your objectives to see if your questions still support them. This is especially important if you’re working on a tight timeline, like when figuring out how to write your thesis in 30 days. Keep these points in mind:
- Regularly review and adjust your questions to fit your objectives.
- Ensure that each question contributes to achieving your overall goals.
- Use your objectives as a checklist to evaluate the relevance of your questions.
Selecting Appropriate Research Design Types
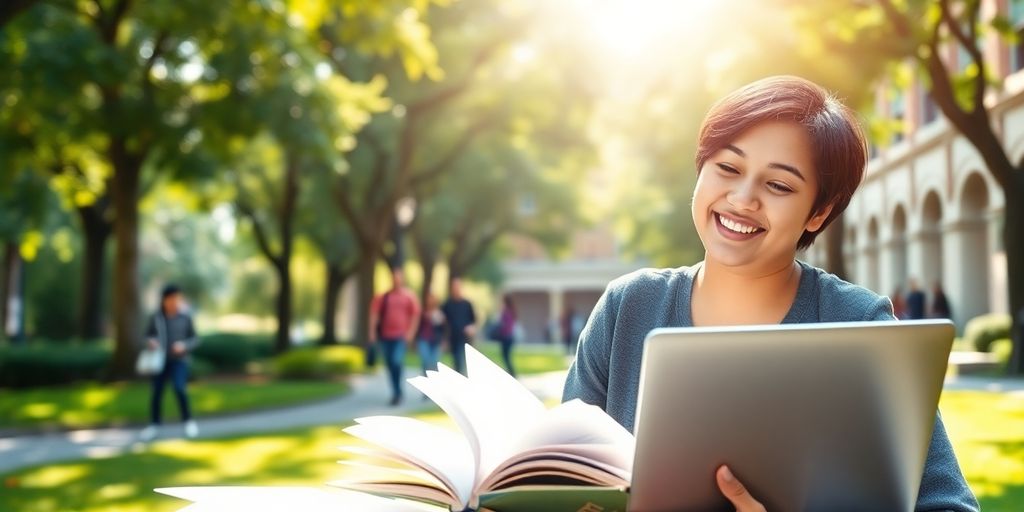
Choosing the right research design is like picking the perfect tool for a job. It’s all about matching the design to your specific research questions and goals. Here, we’ll dive into the main types of research designs you might consider.
Experimental vs. Non-Experimental Designs
When it comes to research, you’ve got two main roads: experimental and non-experimental. Experimental designs are all about control and manipulation. You set up experiments to test cause-and-effect relationships by manipulating variables and observing the outcomes. Think of it like a science lab where you’re the one conducting the tests. On the other hand, non-experimental designs are more observational. You’re not changing anything, just watching and recording what happens naturally. This can be useful when manipulation isn’t possible or ethical.
Quasi-Experimental Design Considerations
Quasi-experimental designs sit somewhere in the middle. They’re like experimental designs but without the full control over variables. This might happen if you can’t randomly assign participants to groups. For example, you might study the effect of a new teaching method in two different schools, but you can’t control which students go to which school. It’s a bit of a compromise, but it allows for real-world application while still trying to infer causality.
Choosing the Right Design for Your Study
Picking the right design depends on a few things:
- Research Question: What are you trying to find out? If you’re looking at cause and effect, experimental might be your best bet.
- Resources: Do you have the time and money for a full-blown experiment? If not, non-experimental might be the way to go.
- Ethics: Sometimes, you just can’t manipulate variables because it wouldn’t be right. In these cases, non-experimental or quasi-experimental designs are more appropriate.
By understanding these options, you can make an informed choice that aligns with your research goals. For more insights on aligning research design with your objectives, check out this guide that covers various types of quantitative research designs and their applications.
Data Collection and Analysis in Causal Research
Methods for Collecting Reliable Data
In causal research, gathering data is a crucial step. You need to decide how to collect data, whether through surveys, interviews, or even digital means like WhatsApp. Each method has its pros and cons, so think about what fits your study best. Surveys can reach a large audience, but interviews might give you deeper insights. Digital tools can offer convenience and real-time data but might miss out on personal nuances.
Analyzing Data for Causal Inference
Once you've gathered your data, the next step is analysis. This is where you determine if there's a causal link between variables. Statistical software can help, but it's important to choose the right tests. For example, regression analysis might be suitable for some studies, while others might require more complex models. The key is to ensure your analysis aligns with your research questions.
Ensuring Data Validity and Reliability
Finally, it's essential to make sure your data is both valid and reliable. Validity means your data accurately reflects what you aim to measure. Reliability means you could get the same results if you repeated the study. To achieve this, consider techniques like triangulation, where you use multiple methods to collect data. This can help identify any inconsistencies and strengthen your findings.
Addressing Ethical and Practical Challenges
Ethical Considerations in Causal Research
When designing causal research, ethical considerations are a key concern. Researchers must prioritize obtaining informed consent from participants, ensuring that they are fully aware of the study's nature and their role in it. Confidentiality is another critical aspect, requiring researchers to safeguard participants' personal information. Additionally, any potential harm or discomfort to participants should be minimized. In the context of ex post facto design research, ethical challenges also arise from analyzing pre-existing data, where issues of privacy and consent can become complex.
Overcoming Practical Limitations
Practical limitations often present significant hurdles in causal research design. These can include limited resources, time constraints, and difficulties in accessing data or participants. Researchers must navigate these challenges by making strategic choices, such as prioritizing certain aspects of the study over others or seeking alternative data sources. A well-thought-out plan can help ensure that the research remains feasible and maintains its integrity. In some cases, leveraging technology or collaborating with other researchers can also provide creative solutions to practical obstacles.
Balancing Scientific Rigor with Feasibility
Achieving a balance between scientific rigor and feasibility is crucial in causal research. While it is important to maintain a high standard of scientific accuracy, researchers must also consider the practical aspects of conducting their studies. This might mean adapting methodologies to fit within budgetary constraints or modifying timelines to accommodate unexpected delays. The ethics review process, especially for internet-based research, often requires researchers to adapt their designs to meet both ethical standards and practical realities. By carefully planning and remaining flexible, researchers can conduct studies that are both ethically sound and practically viable.
Enhancing Research Design with Advanced Tools
Utilizing Technology in Research Design
In today's research landscape, technology plays a key role in shaping how studies are conducted. From data collection to analysis, tech tools streamline processes and make complex tasks more manageable. Tech advancements allow researchers to collect high-quality data efficiently, making it easier to draw meaningful conclusions. For instance, social media platforms like Instagram can be used to gather data on user behavior and preferences, offering insights that were previously hard to obtain.
Incorporating Biosensors and iMotions
Biosensors, like eye trackers and EEG devices, provide researchers with the ability to capture data that goes beyond traditional methods. These tools offer a window into the physiological and emotional states of participants, enabling a deeper understanding of human behavior. iMotions software, in particular, is a powerful tool for integrating data from multiple biosensors, providing a comprehensive view of participant responses. This integration is particularly useful in studies where understanding the interaction between different stimuli and responses is crucial.
Leveraging Software for Data Integration
The use of advanced software solutions is pivotal in handling and integrating large datasets. These tools help in organizing data efficiently, ensuring that researchers can focus on analysis rather than data management. Software solutions provide the framework for combining various data types, making it easier to interpret complex datasets. This is especially important in studies that require the synthesis of qualitative and quantitative data to form a cohesive understanding of the research questions.
Ensuring Validity and Reliability in Causal Research
Internal vs. External Validity
When you're diving into causal research, understanding the difference between internal and external validity is key. Internal validity asks if the study truly shows a cause-and-effect relationship between variables. Imagine you're testing if a new teaching method improves student grades. If your study is internally valid, you can confidently say that any grade changes are due to your method, not some other factor like extra tutoring. On the flip side, external validity is all about generalizability. Can your findings be applied to other settings or populations? If you discover that your teaching method works wonders in one school, external validity checks if it would work just as well elsewhere.
Strategies for Enhancing Reliability
Reliability is about consistency. If you measure something today and again tomorrow, will you get the same result? That's reliability. To boost it, consider these strategies:
- Standardize Procedures: Keep your methods consistent across all participants and trials.
- Use Reliable Instruments: Ensure your tools and measures are tested and proven to give stable results.
- Train Researchers: Make sure everyone involved in data collection is on the same page and knows exactly what to do.
Replicability and Generalizability of Findings
Replicability is like the gold standard in research. If someone else can repeat your study and get the same results, that's a big win for scientific credibility. Generalizability, meanwhile, asks if your findings apply beyond your study's specific context. Say you conducted research on stress and productivity in a tech company. Can those results be applied to other industries or job roles? That's the big question of generalizability. It's crucial to design studies that not only answer your research questions but also hold up when tested by others or in different settings.
When doing causal research, it's super important to make sure your results are both valid and reliable. This means you need to check that your methods are solid and that your findings can be trusted. If you're feeling stuck or unsure about how to tackle your thesis, don't worry! Visit our website for helpful tips and a step-by-step plan to guide you through the process. Let's make your thesis journey easier together!
Conclusion
In wrapping up our exploration of designing causal research, it's clear that a well-thought-out approach is key to obtaining meaningful results. By carefully planning each step—from formulating research questions to selecting the right design and methods—researchers can ensure their studies are both effective and reliable. This guide has aimed to provide a practical framework for those embarking on this journey, emphasizing the importance of clarity and simplicity in research design. With these tools at your disposal, you're well-equipped to conduct research that not only answers your questions but also contributes valuable insights to your field. Remember, the success of your research lies in the details, so take the time to plan thoroughly and execute with precision.
Frequently Asked Questions
What is causal research design?
Causal research design is a way to find out if one thing causes another. It's like a detective solving a mystery by figuring out if one event leads to another.
Why is it important to have clear research questions?
Clear research questions help guide your study. They make sure you know exactly what you're trying to find out, so you don't get lost along the way.
How do you choose the right research design for a study?
Choosing the right research design depends on what you're trying to learn. You need to think about your goals, the questions you're asking, and the tools you have.
What are some methods to collect reliable data?
Reliable data can be collected using surveys, interviews, or experiments. It's important to choose a method that fits your research question and makes sense for your study.
What challenges might you face in causal research?
In causal research, you might face challenges like ethical issues, limited resources, or difficulty in controlling all the variables.
How can technology help in research design?
Technology can help by providing tools to collect and analyze data more efficiently. It makes the research process faster and often more accurate.
The Ultimate Guide to Designing Causal Research That Gets Results

So you're thinking about diving into causal research design, huh? It's like figuring out a puzzle, but with science. This guide is all about helping you piece it together. Whether you're a newbie or a seasoned pro, understanding the basics and getting the right tools is key. From setting your research questions to tackling ethical dilemmas, we've got it all covered. Let's make sure your research doesn't just sit on a shelf but actually gets results.
Key Takeaways
- Get clear on what causal research design really means and why it matters.
- Crafting solid research questions and hypotheses is the backbone of any study.
- Picking the right research design type can make or break your project.
- Collecting and analyzing data is where the magic happens, but it needs to be done right.
- Don't ignore the ethical and practical challenges; they can derail your research if not handled.
Understanding the Foundations of Causal Research Design
Defining Causal Research Design
Causal research design is all about figuring out the cause-and-effect relationships between variables. Imagine you're trying to see if drinking coffee makes people more alert. That's what causal research aims to do—determine if one thing causes another. It's a big deal because it helps in making predictions and understanding the world better. Causal research design is crucial because it allows researchers to make informed decisions based on evidence. Unlike correlational studies, which only show that two things are related, causal research digs deeper to find out why and how they're connected.
Historical Context and Evolution
The journey of causal research design is pretty fascinating. It started centuries ago when scholars began questioning the "why" behind natural phenomena. Over time, this curiosity evolved into structured methods for testing hypotheses. In the last century, the development of experimental and quasi-experimental designs has been pivotal in refining these methods. Today, causal research is more sophisticated, thanks to technological advancements and effective research design principles. These developments have made it easier to replicate studies and ensure that findings are reliable and valid.
Key Principles and Concepts
At the heart of causal research design are some key principles. First, there's the idea of manipulation—changing one variable to see what happens to another. Next is control, where researchers try to keep everything else constant to ensure that any changes in the outcome are due to the manipulated variable. Randomization is another biggie, helping to minimize biases and ensure that results are not skewed by other factors. These principles are essential for maintaining the integrity of the research and ensuring that the results are trustworthy.
In summary, understanding the foundations of causal research design is about knowing how to set up a study that can truly test cause-and-effect relationships. It's about being meticulous and thoughtful in every step, from defining the research question to choosing the right method. With a solid grasp of these concepts, researchers can produce findings that are not only insightful but also reliable and applicable in real-world scenarios.
Formulating Research Questions and Hypotheses
Crafting Precise Research Questions
Crafting precise research questions is the cornerstone of any successful study. These questions guide the entire research process, helping to focus efforts and resources on the most relevant aspects. To formulate a research question, start by identifying the problem or topic of interest. Break it down into smaller, manageable parts to clarify what you need to investigate. This process is similar to how one might approach AI's value creation, where philosophical frameworks guide learning processes. Consider the following steps:
- Identify the broad topic or issue you want to explore.
- Narrow it down to specific aspects that are feasible to study.
- Frame your question in a way that is clear, focused, and researchable.
Developing Testable Hypotheses
Once you have a clear research question, the next step is to develop a hypothesis. A hypothesis is a testable statement that predicts the relationship between variables. It should stem from your research question and be informed by existing literature. For example, if your research question is about the impact of social media on job search success, you might hypothesize that "LinkedIn usage increases job acquisition rates." Here’s how to write your hypothesis:
- Clearly state the expected relationship between variables.
- Ensure it is testable with the available methods.
- Align it with the research design and objectives.
Aligning Questions with Research Objectives
Aligning your research questions with your objectives ensures that your study remains focused and relevant. This alignment helps in maintaining a clear trajectory throughout your research process. To achieve this, regularly revisit your objectives to see if your questions still support them. This is especially important if you’re working on a tight timeline, like when figuring out how to write your thesis in 30 days. Keep these points in mind:
- Regularly review and adjust your questions to fit your objectives.
- Ensure that each question contributes to achieving your overall goals.
- Use your objectives as a checklist to evaluate the relevance of your questions.
Selecting Appropriate Research Design Types
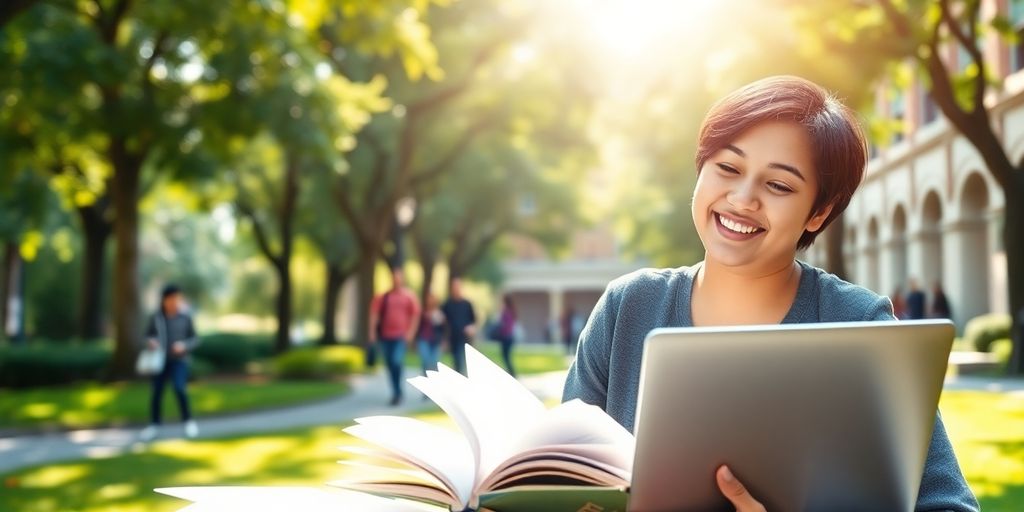
Choosing the right research design is like picking the perfect tool for a job. It’s all about matching the design to your specific research questions and goals. Here, we’ll dive into the main types of research designs you might consider.
Experimental vs. Non-Experimental Designs
When it comes to research, you’ve got two main roads: experimental and non-experimental. Experimental designs are all about control and manipulation. You set up experiments to test cause-and-effect relationships by manipulating variables and observing the outcomes. Think of it like a science lab where you’re the one conducting the tests. On the other hand, non-experimental designs are more observational. You’re not changing anything, just watching and recording what happens naturally. This can be useful when manipulation isn’t possible or ethical.
Quasi-Experimental Design Considerations
Quasi-experimental designs sit somewhere in the middle. They’re like experimental designs but without the full control over variables. This might happen if you can’t randomly assign participants to groups. For example, you might study the effect of a new teaching method in two different schools, but you can’t control which students go to which school. It’s a bit of a compromise, but it allows for real-world application while still trying to infer causality.
Choosing the Right Design for Your Study
Picking the right design depends on a few things:
- Research Question: What are you trying to find out? If you’re looking at cause and effect, experimental might be your best bet.
- Resources: Do you have the time and money for a full-blown experiment? If not, non-experimental might be the way to go.
- Ethics: Sometimes, you just can’t manipulate variables because it wouldn’t be right. In these cases, non-experimental or quasi-experimental designs are more appropriate.
By understanding these options, you can make an informed choice that aligns with your research goals. For more insights on aligning research design with your objectives, check out this guide that covers various types of quantitative research designs and their applications.
Data Collection and Analysis in Causal Research
Methods for Collecting Reliable Data
In causal research, gathering data is a crucial step. You need to decide how to collect data, whether through surveys, interviews, or even digital means like WhatsApp. Each method has its pros and cons, so think about what fits your study best. Surveys can reach a large audience, but interviews might give you deeper insights. Digital tools can offer convenience and real-time data but might miss out on personal nuances.
Analyzing Data for Causal Inference
Once you've gathered your data, the next step is analysis. This is where you determine if there's a causal link between variables. Statistical software can help, but it's important to choose the right tests. For example, regression analysis might be suitable for some studies, while others might require more complex models. The key is to ensure your analysis aligns with your research questions.
Ensuring Data Validity and Reliability
Finally, it's essential to make sure your data is both valid and reliable. Validity means your data accurately reflects what you aim to measure. Reliability means you could get the same results if you repeated the study. To achieve this, consider techniques like triangulation, where you use multiple methods to collect data. This can help identify any inconsistencies and strengthen your findings.
Addressing Ethical and Practical Challenges
Ethical Considerations in Causal Research
When designing causal research, ethical considerations are a key concern. Researchers must prioritize obtaining informed consent from participants, ensuring that they are fully aware of the study's nature and their role in it. Confidentiality is another critical aspect, requiring researchers to safeguard participants' personal information. Additionally, any potential harm or discomfort to participants should be minimized. In the context of ex post facto design research, ethical challenges also arise from analyzing pre-existing data, where issues of privacy and consent can become complex.
Overcoming Practical Limitations
Practical limitations often present significant hurdles in causal research design. These can include limited resources, time constraints, and difficulties in accessing data or participants. Researchers must navigate these challenges by making strategic choices, such as prioritizing certain aspects of the study over others or seeking alternative data sources. A well-thought-out plan can help ensure that the research remains feasible and maintains its integrity. In some cases, leveraging technology or collaborating with other researchers can also provide creative solutions to practical obstacles.
Balancing Scientific Rigor with Feasibility
Achieving a balance between scientific rigor and feasibility is crucial in causal research. While it is important to maintain a high standard of scientific accuracy, researchers must also consider the practical aspects of conducting their studies. This might mean adapting methodologies to fit within budgetary constraints or modifying timelines to accommodate unexpected delays. The ethics review process, especially for internet-based research, often requires researchers to adapt their designs to meet both ethical standards and practical realities. By carefully planning and remaining flexible, researchers can conduct studies that are both ethically sound and practically viable.
Enhancing Research Design with Advanced Tools
Utilizing Technology in Research Design
In today's research landscape, technology plays a key role in shaping how studies are conducted. From data collection to analysis, tech tools streamline processes and make complex tasks more manageable. Tech advancements allow researchers to collect high-quality data efficiently, making it easier to draw meaningful conclusions. For instance, social media platforms like Instagram can be used to gather data on user behavior and preferences, offering insights that were previously hard to obtain.
Incorporating Biosensors and iMotions
Biosensors, like eye trackers and EEG devices, provide researchers with the ability to capture data that goes beyond traditional methods. These tools offer a window into the physiological and emotional states of participants, enabling a deeper understanding of human behavior. iMotions software, in particular, is a powerful tool for integrating data from multiple biosensors, providing a comprehensive view of participant responses. This integration is particularly useful in studies where understanding the interaction between different stimuli and responses is crucial.
Leveraging Software for Data Integration
The use of advanced software solutions is pivotal in handling and integrating large datasets. These tools help in organizing data efficiently, ensuring that researchers can focus on analysis rather than data management. Software solutions provide the framework for combining various data types, making it easier to interpret complex datasets. This is especially important in studies that require the synthesis of qualitative and quantitative data to form a cohesive understanding of the research questions.
Ensuring Validity and Reliability in Causal Research
Internal vs. External Validity
When you're diving into causal research, understanding the difference between internal and external validity is key. Internal validity asks if the study truly shows a cause-and-effect relationship between variables. Imagine you're testing if a new teaching method improves student grades. If your study is internally valid, you can confidently say that any grade changes are due to your method, not some other factor like extra tutoring. On the flip side, external validity is all about generalizability. Can your findings be applied to other settings or populations? If you discover that your teaching method works wonders in one school, external validity checks if it would work just as well elsewhere.
Strategies for Enhancing Reliability
Reliability is about consistency. If you measure something today and again tomorrow, will you get the same result? That's reliability. To boost it, consider these strategies:
- Standardize Procedures: Keep your methods consistent across all participants and trials.
- Use Reliable Instruments: Ensure your tools and measures are tested and proven to give stable results.
- Train Researchers: Make sure everyone involved in data collection is on the same page and knows exactly what to do.
Replicability and Generalizability of Findings
Replicability is like the gold standard in research. If someone else can repeat your study and get the same results, that's a big win for scientific credibility. Generalizability, meanwhile, asks if your findings apply beyond your study's specific context. Say you conducted research on stress and productivity in a tech company. Can those results be applied to other industries or job roles? That's the big question of generalizability. It's crucial to design studies that not only answer your research questions but also hold up when tested by others or in different settings.
When doing causal research, it's super important to make sure your results are both valid and reliable. This means you need to check that your methods are solid and that your findings can be trusted. If you're feeling stuck or unsure about how to tackle your thesis, don't worry! Visit our website for helpful tips and a step-by-step plan to guide you through the process. Let's make your thesis journey easier together!
Conclusion
In wrapping up our exploration of designing causal research, it's clear that a well-thought-out approach is key to obtaining meaningful results. By carefully planning each step—from formulating research questions to selecting the right design and methods—researchers can ensure their studies are both effective and reliable. This guide has aimed to provide a practical framework for those embarking on this journey, emphasizing the importance of clarity and simplicity in research design. With these tools at your disposal, you're well-equipped to conduct research that not only answers your questions but also contributes valuable insights to your field. Remember, the success of your research lies in the details, so take the time to plan thoroughly and execute with precision.
Frequently Asked Questions
What is causal research design?
Causal research design is a way to find out if one thing causes another. It's like a detective solving a mystery by figuring out if one event leads to another.
Why is it important to have clear research questions?
Clear research questions help guide your study. They make sure you know exactly what you're trying to find out, so you don't get lost along the way.
How do you choose the right research design for a study?
Choosing the right research design depends on what you're trying to learn. You need to think about your goals, the questions you're asking, and the tools you have.
What are some methods to collect reliable data?
Reliable data can be collected using surveys, interviews, or experiments. It's important to choose a method that fits your research question and makes sense for your study.
What challenges might you face in causal research?
In causal research, you might face challenges like ethical issues, limited resources, or difficulty in controlling all the variables.
How can technology help in research design?
Technology can help by providing tools to collect and analyze data more efficiently. It makes the research process faster and often more accurate.